Getting familiar with conjoint analysis
Conjoint-Analysis asks respondents to make a choice between multiple sets of criteria in a real-world scenario to help researchers understand which features are prioritized and considered during the decision-making phase.
What is Conjoint Analysis?
Conjoint-analysis is a quantitative research method that asks respondents to make selections between groups of attributes in a scenario against alternative scenarios made up of different attributes. It is considered more predictive of a buyer’s purchase behavior as it does not let respondents cherry-pick attributes or select the “ideal state” of a product in isolation. Instead, respondents are given sets of features in a product or package where they make trade-offs, indicating which features are most important and what they value most. Conjoint analysis survey questions are often used to evaluate features in products, pricing for packages, and different product offerings by a business.
Choice-based Conjoint-Analysis
Although there are several kinds of conjoint-analysis, the most commonly used technique is a choice-based conjoint analysis, which is used in many real-life purchasing scenarios.
Rather than asking respondents to give their opinion about an isolated event, conjoint analysis allows you to measure two (or more) scenarios at a time against the alternatives available. Respondents are asked to make trade-offs between the presented scenarios, rather than choosing or customizing specific options. Each scenario includes a complete set of attributes or features to provide context that may influence their decision to choose one scenario over another. For example, respondents being asked to compare internet service packages against another based on what each package offers.
These answers give researchers additional context about what is most important to their respondents when evaluating a complete package, rather than individual characteristics.
An example of a conjoint analysis might be asking consumers to compare features of internet cable packages, then compare the packages overall.
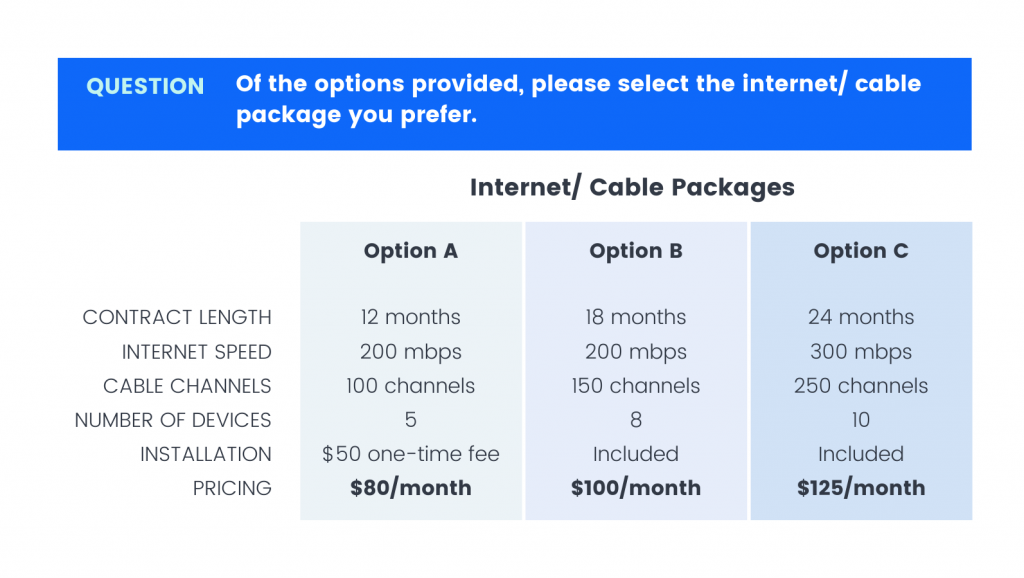
A discrete choice conjoint analysis allows respondents to select between a series of products, or choose “none” which is considered more indicative of real-world behavior. For our example, if the respondent doesn’t see value in any of the presented options, they may choose not to buy an internet package from this company at all.
Adaptive conjoint analysis
Another type of conjoint analysis is an Adaptive Conjoint Analysis, which requires software to use previous responses from respondents to tailor the questionnaire. Although it follows the same structure of asking respondents to make trade-offs to determine weights and information, it ultimately can result in smaller or more in-depth surveys about specific attributes. Adaptive Conjoint Analysis relies on a type of branching logic that eliminates attributes that are less relevant to the respondent and keeps their focus on fewer attributes throughout the survey.
Weighting in conjoint analysis
Conjoint Analysis question options apply weights to the attributes in a grouping to elevate their importance. Weights are determined through a linear regression model that asks respondents to go through a series of configurations of a product or package. Using experimental design, these are then analyzed and weights are determined for the individual attributes.
The values of the individual attributes that indicate the respondents’ trade-offs in a product are called part-worths, and they are used in determining the weight of each attribute in the grouping.
In the internet package example, if “internet speed” was the most important attribute to respondents, it would be given a greater weight to indicate its significance in the respondent’s decision to choose this package. Alternatively, the contract length may be considered a trade-off for respondents wanting faster internet speeds, so the weight applied to “contract” would be given a weight that balances the “internet speed” attribute and makes the trade-off more comparable. These influence the choice the respondent makes and lets researchers discover the right balance between attributes offered in a conjoint selection.
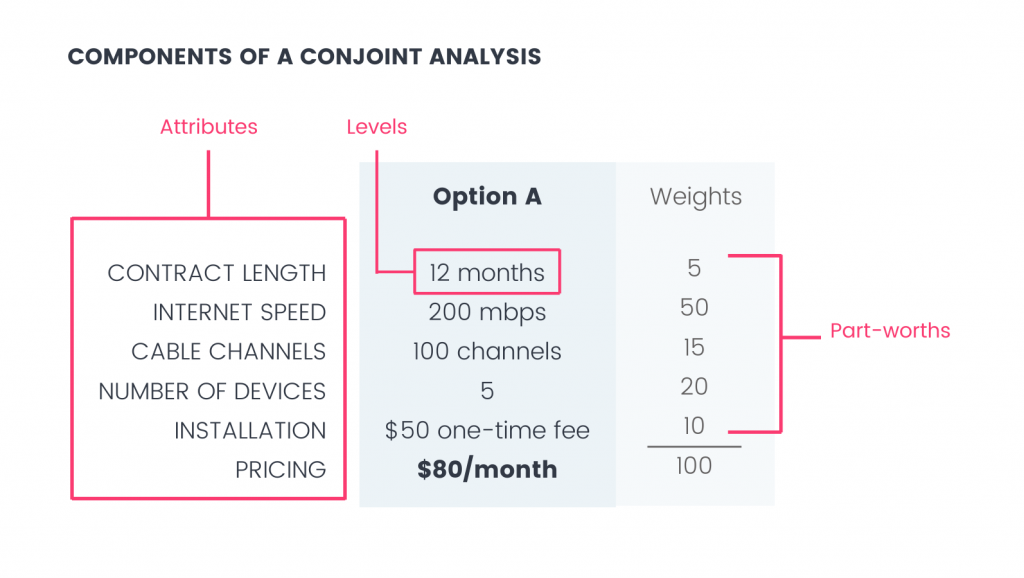
Conjoint analysis terms and vocabulary
- Adaptive Conjoint Analysis: A digitally based conjoint analysis test that uses an algorithm specific to the respondent’s answers to tailor the questions to attributes that are relevant to the respondent.
- Attributes (Features): The criteria that make up a complete set of information to be compared in a conjoint analysis. In our internet packages example, the attributes are “Contract Length”, “Internet speed”, “channels provided”, etc.
- Choice-based conjoint Analysis: The most common type of conjoint-analysis that asks respondents to make a choice between two or more offerings made of a set of weighted attributes.
- Discrete-choice conjoint analysis: A choice-based conjoint analysis that provides a “none of the above” option in addition to a series of options. It most clearly matches real-world purchase decisions by giving respondents the option not to select any of the choices.
- Experimental Design: A psychological practice of research design that refers to the distribution of respondents in different parts of an experiment. It often includes at least one experimental group and one control group.
- Full-profile: A conjoint analysis that explores all of the attributes.
- Levels: Categories of specific attributes. In our internet packages example, levels for the attribute “Contract Length” are “12 months”, “18 months”, and “24 months.”
- Part-worths (Utilities): The partial value of a complete product, divided among its attributes to assign value to each part.
- Partial-Profile: A conjoint analysis that only explores a few attributes at a time.
Conjoint analysis offers greater depth into consumer purchasing decisions through weighting and assessing the data to see which features bring greater value to products. These insights can then be used to create new or better products, special offers, or inform competitive market opportunities.
Do you want to distribute your survey? Pollfish offers you access to millions of targeted consumers to get survey responses from $0.95 per complete. Launch your survey today.