Organic Random Device Engagement For Better Survey Research
8 Ways Survey Research is Better with Organic Random Device Engagement
This is an excerpt from a recent academic paper written by Dr. David Rothschild, Economist at Microsoft Research & Dr. Tobias Konitzer, C.S.O. and co-founder of PredictWise.
Organic random device engagement (RDE) polling relies on advertising networks, or other portals on devices, to engage random people where they are. One of the most common versions of this is within advertising modules on smartphones, but it can easily be placed in gaming, virtual reality, etc.
Survey respondents are asked to participate in a poll in exchange for an incentive token that stays true to the philosophy of the app in which they are organically engaged. This method has a number of advantages:
1. Fast
RDE can be extremely fast. RDD takes days (and weeks in some cases). Using social networks (assisted crowdsourcing) can be done a little faster, but still lacks speed compared to RDE. Using online panels is comparable in speed, if you pay for extra respondents from a merged panel (online panels will charge extra to get respondents from other panels to increase speed).
2. Cost-effective
RDE is extremely inexpensive compared with other sampling 12 options. The major RDE providers, like Pollfish, Dalia or Tap Research, charge 10% the cost of RDD, 20% the cost of using assisted crowdsourcing, and 25% the cost of online panels.
3. Coverage is good and growing
Accuracy is good because coverage is good. And, while RDE is still behind RDD in coverage at this time, it will reach parity soon. Coverage is similar to social media-based assisted crowdsource polling and much better than with online panels. Online panels have a very small footprint, which also affects their ability to get depth in population.
4. Response rate is solid
Pollfish reports a reasonable response rate (much higher than RDD), conditional on being targeted for a poll (to completion of the survey, that is). Online panels have low sign-up rates and high drop out but do not post comparable response rates. Social media-based polling, in assisted crowdsourcing, is reliant on ads that suffer from a very low click-through.
5. Flexible
RDE is meant to be flexible with the growth of devices. It should provide a seamless experience across device types. RDD is stuck with telephones, by definition. And, RDD is subject to interviewer effects (albeit to a smaller extent than in-person surveys), meaning that tone of voice can influence considerations of the respondent, or trigger undesired interviewer respondent interactions, ultimately introducing measurement error. RDE, with its streamlined experience, is not subject to this kind of error. (Tucker 1983; West and Blom 2017)
6. Telemetry data
RDE is able to supplement collected attitudinal data with a rich array of para or telemetry data. As we know, people who answer surveys are fundamentally different than people who do not. As the progressive analytics shop, CIVIS has argued recently, a battery of nearly 30 additional demographic, attitudinal, and lifestyle questions that get at notions of social trust and cosmopolitanism is necessary to be able to weight and correct for all the ways in which survey respondents are unusual. As Konitzer, Eckman and Rothschild (2016) argue, telemetry data is a much more cost-effective (and unobtrusive) way to collect these variables. Home and work location, commuting or mobility patterns or the political makeup of one's neighborhood or social network, derived from satellite-based (read: extremely accurate) longitudinal location-coordinate data predict demographic variables well, such as race and income. And, applications on the device can more accurately describe political traits prone to erroneous self-report, such as frequency of political discussion, political engagement or knowledge.
7. RDE will get stronger in the future
Penetration of devices will further increase in the future, increasing reach of RDE in the US, and making RDE the only viable alternatives in less developed markets. But the rosy future for RDE is not just about penetration. Advances in bridging Ad IDs with other known identifiers in the American market, such as voter file IDs, Experian Gold IDs, etc., mean that individual targeting based on financial history or credit card spending patterns will be possible. And, RDE will be able to adopt list-based polling, in which political survey firms poll directly from the voter file, large-scale administrative data detailing the turnout and registration history of 250,000,000 Americans.
8. River sampling is different, as devices are unknown
River sampling can either mean banner-ad based polling or engagement with respondents via legacy websites or similar places RDE recruits from. In contrast to RDE, devices are unknown to river samplers: River sampling usually does not have access to the Ad ID, introducing two huge disadvantages: River samples have no way to address SUMA it is possible for fraudsters to engage with the same poll twice to increase chances to win the price for participation, especially if it comes in the form of financial incentives. And, any degree of demographic/geographic (not to mention individual) targeting is virtually impossible. In addition, banner ads themselves, similar to social-media ads, suffer from disastrous response rates. Good RDE polling is done with the cooperation of the publisher, providing a native experience, while banners ads are pushed through the ad-network. This degraded user experience depresses response rates and can introduce serious measurement error.
Second, ad-networks optimize their delivery in a way that fights against the random sample. The users are chosen because they are more likely to respond, due to unobserved variables (at least to the survey researcher), that are correlated with how they will respond. As this underlying data is never shared, it is impossible to correct for by the survey researcher.
However, just like every other modern online survey sampling method (RDD, assisted crowdsourcing, online panels), RDE relies on non-probability sampling. There is no sample method (anymore) that has perfect coverage and known probabilities for any respondent. This is one of the reasons we have developed analytics to overcome known biases. And, RDE has bias that we understand and can overcome, and additional data points that add to the power of correcting bias, such as telemetry data that is not available to RDD. While RDD has shifting and shrinking coverage, online panels suffer from panel fatigue and panel conditioning, and assisted crowdsourcing has survey bias introduced by efficient but to the polling firm nontransparent targeting algorithms that cannot be addressed, RDE is our method of choice, and the future, in the ever-changing market of polling.
Examples of RDE
Here we review work published in both Goel, Obeng and Rothschild (2015) and Konitzer, Corbett-Davies and Rothschild (N.d.) to showcase how effective RDE samples can be. And, add examples from the 2017-2018 special congressional elections.
Example 1:
(Goel, Obeng and Rothschild 2015) shows how RDE, through Pollfish, is able to closely match gold-standard polling such as the General Social Survey. This gold-standard uses yet another method: house-calls. This is unaffordable for most research, so we have left it off of this paper, but it provides a useful benchmark.
Example 2:
(Konitzer, Corbett-Davies and Rothschild N.d.) shows how RDE, utilizing the Pollfish platform, is able to closely match RDD polling in the 2016 election (actually doing slightly better). This is an example of using RDE samples with an analytic method call Dynamic MRP. The analytics methods are detailed in their paper.
When (Konitzer, Corbett-Davies and Rothschild N.d.) quantifies their state-by-state errors, they show that their predictions based on a single poll are not significantly worse than the predictions from poll aggregators. They compare their state-by-state estimates against the actual outcome. Compared to poll aggregator Huffington Post Pollster, their Root Mean Squared Error (RMSE) is only slightly higher: 4.24 percentage points vs. 3.62 percentage points (for 50 states excluding DC).
When they focus on the 15 closest states, predictive accuracy is even higher. The RMSE is 2.89 percentage points, compared to 2.57 percentage points of Huffington Post Pollster. Overall, besides binary accuracy the RDE-based polling predictions also have a low error in the precise percentage value.
This is illustrated in Figure 1.
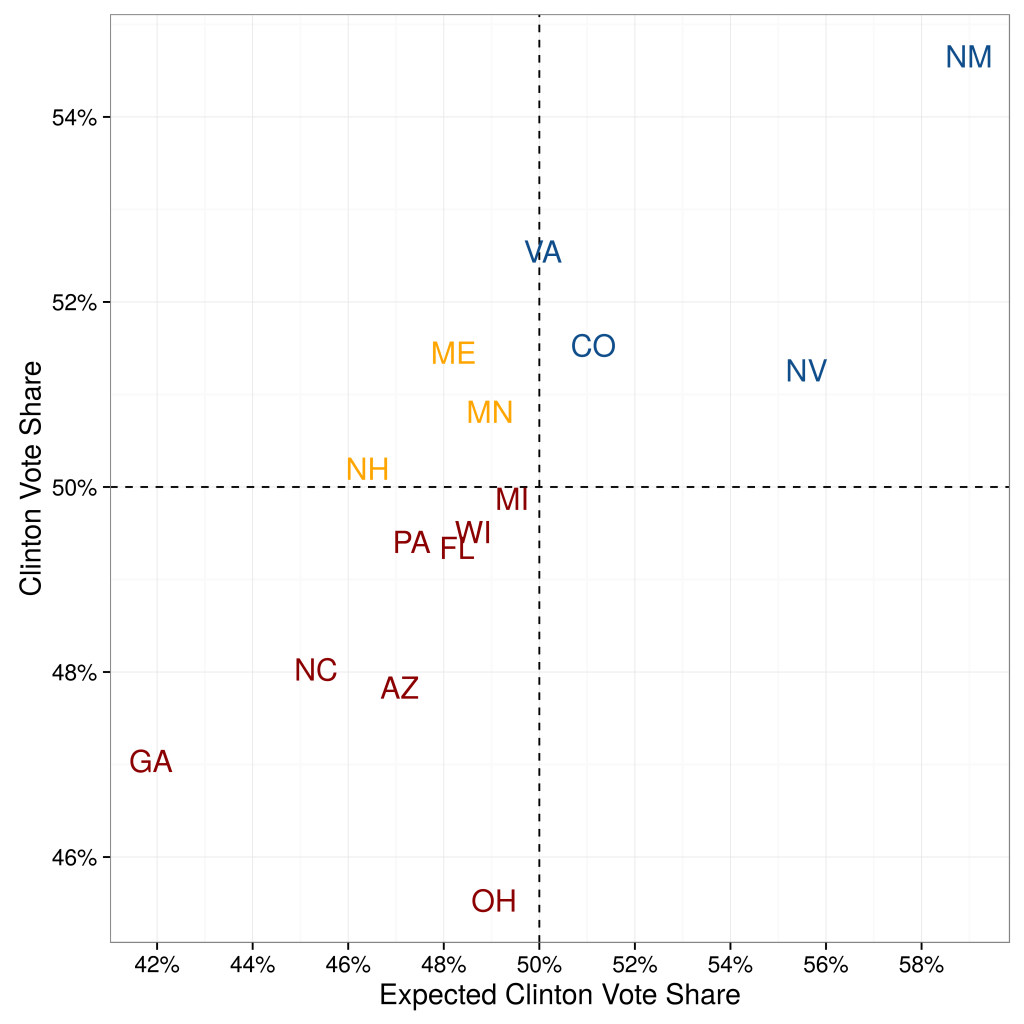
Not only are RDE-based polling state-by-state estimations fairly accurate, they also add meaningful signal to the poll aggregations. The left panel of Figure 2 displays the correlation between state-by-state errors of our predictions and the state-by-state errors of Huffington Post Pollster, and the right panel compares the distribution of errors across their approach and Huffington Post Pollster. At the very least, using RDE has significant potential to increase the quality of aggregators, as we discuss more below.
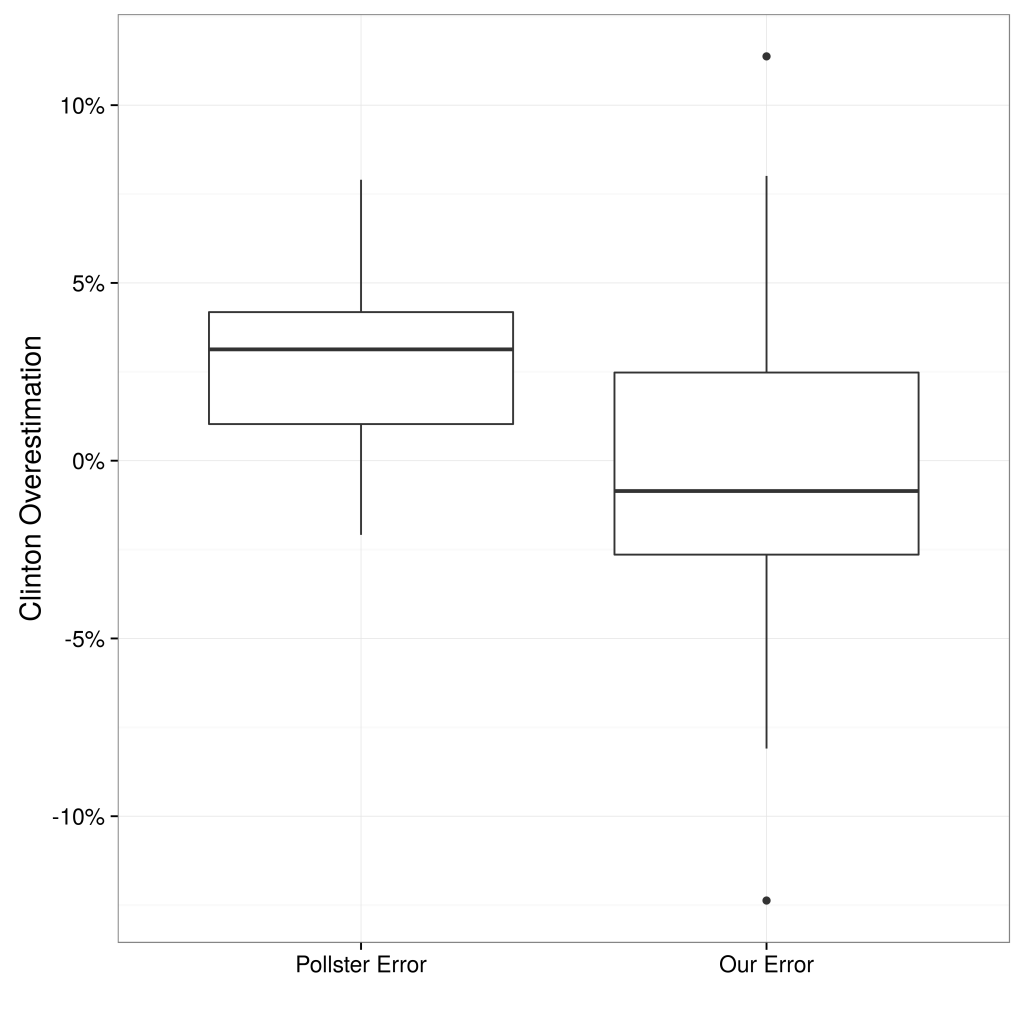
Example 3:
During the course of 2017 and 2018 polling firms have employed all three new methods in predicting Congressional election outcomes: RDE comes out way above the other two.
In this paper we outlined four methods of data collection for surveys. The first method, Random Digit Dialing (RDD), is the traditional method, working fine, but it is doomed in the next few years. Thus, the paper is really about which of the new online survey sampling methods will replace it: online panels, Assisted Crowdsourcing, or Random Device Engagment (RDE). We believe strongly that RDE is the future.
River Sampling Versus RDE Sampling: Which is Superior for Market Research
River Sampling Versus RDE Sampling: Which is Superior for Market Research
River sampling versus RDE (Random Device Engagement) sampling: it’s a showdown for the ages. As two of the foremost players in survey sampling methods, these two always appear to compete head-to-head for the attention and execution of market researchers.
As prominent players in the survey research sector, both the RDE and river sampling methods are considered superior to using survey panels for market research.
As the two dominant means of obtaining a survey sample, which forms the core of any market research campaign, it is crucial to be diligent when deliberating over which method to use for your survey sampling.
This article posits river sampling and RDE sampling in a showdown, so that market researchers and general researchers comprehend which is more fitting for their market research needs.
Defining River Sampling
River sampling is an online survey sampling method — the earliest and simplest of its kind. This non-probability sampling method obtains survey respondents by requesting online visitors to take a survey via clicking on a link that routes them to the survey.
The link is placed somewhere in a webpage, email or another area in the digital space. Typically, respondents are scouted through web elements such as banners, ads, promotions and offers.
When site or app users click on the link used in river sampling, they are first routed to the screener portion of the survey and if they fit the requirements set in the screener, they are then routed to the questionnaire portion.
River sampling derives its name from the metaphorical idea that researchers net their study subjects by catching them in the river that is the internet, specifically the flow of traffic in a website.
Also called intercept sampling and real‐time sampling, this method extracts respondents by engaging them while they take part in some other digital activity.
The Two Types of River Sampling
River sampling exists in two forms. While they may appear to be vaguely interchangeable, each form includes a unique method for procuring respondents. In the showdown of river sampling versus RDE sampling, it’s important to understand the workings of each.
Stratified River Sampling
This kind of river sampling involves drawing samples in real-time from online promotions, those that are disseminated through banners, ads, pop-ups and hyperlinks. Market researchers would choose the websites for survey deployment based on statistics on such websites’ traffic.
Convenience River Sampling
This submethod of river sampling involves the placement of promotions and hyperlinks across websites without previously analyzing the websites’ traffic numbers and types of visitors. As such, market researchers deploy surveys in a completely blind manner. The point of this form of sampling is to derive maximum data at a minimum cost.
The Pros and Cons of River Sampling
A commonly used method of sampling respondents, river sampling has several advantages and disadvantages. Understanding them is important for researchers, should they consider using this method, or learning how it differs from other sampling methods, such as RDE sampling.
The Advantages
- Serves as a powerful replacement for survey panels by providing new respondents, those that have not been influenced or conditioned to take part in a survey.
- Engages users in their natural digital environments.
- Its survey callouts/ links exist in easily noticeable digital properties.
- Creates a faster alternative to the focus group, which involves a group discussion where dominant participants can take charge and make it difficult for more demure participants.
- Ensures complete anonymity of respondents.
- Exists as a simple method of data gathering, since all researchers need to do is wait for the data to be aggregated.
- An inexpensive source of sampling.
- A flexible method that collects respondents in the moment, rather than being profiled prior to the survey and recruited manually.
The Disadvantages
- The devices used by potential and opted-in respondents are completely unknown.
- There is no access to an advertisement’s ID.
- Fraudsters can therefore take the same survey twice or more to increase their incentives or the chance to win a prize.
- No degree of demographic, geographic or individual targeting is possible.
- Banner ads generally have insufficient response rates.
- Banners are pushed through ad networks, diminishing the user experience.
- Ad-networks optimize their delivery by fighting against random sampling.
- As such, users are picked due to a higher likelihood of responding, from unobserved variables (to the researcher) correlated with how they will respond. At any rate, none of the data is shared, so it is impossible to correct.
- It is difficult to reach an acceptable level of representation, as respondents are not tracked.
- Surveyors have no inkling of who will participate in the surveys due to the lack of tracking and profiling.
- This method is prone to receive straight-lining from the respondents.
Defining RDE Sampling
RDE sampling, also known as Random Device Engagement is an advanced method of non-probability sampling, one that falls in diametrical opposition with survey panels. It is completely random and organic, with no pre-recruitment and no website monitoring.
RDE sampling refers to the sampling practice of engaging online users on all the devices they are already using, be it within advertising networks, mobile apps and other portals on various devices.
This involves the careful placement of surveys in gaming interfaces and virtual reality, allowing market researchers to offer non-monetary incentives to respondents. These include coins or points in a game, or the ability to win a major virtual in-game prize.
RDE sampling can be disseminated through digital elements similar to the ones used in river sampling, such as banners, ads and other positions on a webpages, such as buttons. These survey callouts e.t. al., must be placed strategically, so that respondents can easily spot them. They must also be created in a way that sparks the curiosity or interest of the webpage’s visitors to click on them in the first place.
RDE engages potential respondents in their natural digital environments and respondents enter the survey voluntarily. This method also ensures complete randomization, as no pre-recruiting efforts are involved.
Respondents are also completely anonymous, in terms of their identities, thus, there is no pressure to answer questions in a particular way, such as one that adheres to societal norms and expectations.
The Key Differences Between River Sampling and RDE Sampling
Many of the traits in RDE sampling render this method to seemingly mimic river sampling, with no apparent distinguishing features. But this is false — there are several ways in which RDE sampling diverges from the river sampling method.
Unlike river sampling, RDE sampling offers a monitoring functionality, which tracks the unique identifier of respondents’ devices. The survey software that carries out RDE sampling works natively with the device when it is optimized correctly. For example, a strong example of this would be a mobile-first survey platform.
Furthermore, unlike river sampling, in which respondents are not tracked or identified by demographics, etc., RDE tracks respondents through a unique ID, one that notifies the researchers when the same respondents are changing devices.
RDE also relies on artificial intelligence to weed out poor quality responses, such as gibberish answers and users who are on a VPN.
The Pros and Cons of RDE Sampling
Random Device Engagement sampling carries various benefits and drawbacks that all market researchers should be aware of, even if they do not choose this sampling method. That is because it is critical to weigh these advantages and disadvantages against those of river sampling for a true comparison.
The Advantages
- A higher quality of data due to AI functionality and automatic quality checks.
- Respondents are not conditioned through pre recruiting tactics or pressured to answer questions in a certain way due to being in their natural digital environments.
- Offers various telemetry data prone to bias correction, involving location history and application usage.
- Has a high coverage due to the heavy usage of mobile phones; phones carry a high penetration of about 70% and decent response rates.
- Avoids fraud from SUMAs (single users on multiple accounts); respondents can only answer once and therefore VPN respondents are disqualified.
- Tracks different devices that respondents use, important given the uncertainty of the future use of phones.
- RDE is fast and cost-effective.
- Able to supplement attitudinal data with a vast array of para or telemetry data.
- For example, those who partake in surveys are rather different than those who don’t. As such, it is necessary to get roughly 30 more demographic, attitudinal, and lifestyle questions to understand social trust and how survey respondents are unusual.
The Disadvantages
- Given that this method involves tracking location and application usage, it is not as anonymous.
- In reference to Point 1, the researchers will have to add the necessary disclaimers to their surveys.
- Surveys on RDE networks may not exist in as diverse a set as they do in river sampling.
- Is still prone to several kinds of survey bias.
- Does not offer perfect coverage or known probabilities for every respondent.
- Respondents may be subject to survey fatigue if the survey is too long and not built with best practices.
The Verdict of the River Sampling Vs. RDE Sampling Showdown
In conclusion, who comes up victorious in the showdown between river sampling and Random Device Engagement sampling? In the spirit of remaining unbiased, the true victor is up to the market researcher, the business owner, or the marketing department of a business.
This is because each business and operation will envision their market research campaigns differently and thus will have different requirements and standards for their campaigns. This includes how they will execute survey sampling.
Both methods secure the privacy of respondents, as respondents are never matched with their identities. However, the major point of difference between these two methods is that river sampling does not capture the devices and app usage of the respondents, while RDE does, given that it tracks respondents through unique IDs.
Thus, when using either of these sampling methods, they will have to be dealt with differently.
In regards to this, market researchers can make the judgment of which sampling method is best. We believe that based on the better data quality and representativeness of the sample, RDE is the superior survey sampling method.
With river sampling, researchers must assess the stability, span and relevance of the promotions used in tandem with the surveys. Additionally, market researchers will need to check security and quota controls during the sampling process. SIngle users participating multiple times must be thwarted with specialized software.
With RDE sampling, the online survey platform must cooperate with the publishers and their networks, so that market researchers can design a native experience with surveys on their platforms. (In river sampling, banner ads are pushed through the ad network instead). Thus, RDE sampling is objectively the stronger sampling method, given that it seamlessly prevents fraud and poor data from its capabilities of tracking down device usage and fending off VPNs, users take part more than once and other nefarious behaviors that mar the data and sample collection process.
Frequently asked questions
What is river sampling?
River sampling is an online survey sampling method that obtains survey respondents by requesting online visitors to take a survey via clicking on a link that routes them to the survey. It is entirely random and organic, with no website monitoring. RDE sampling, on the other hand, refers to the sampling practice of engaging online users on all the devices they are already using, be it within advertising networks, mobile apps, and other portals on various devices.
What is RDE sampling?
Objectively speaking, RDE sampling is better as it provides a more robust sampling method given that it integrates well with AI to prevent instances of fraud and insufficient data. It also tracks device usage and can notify researchers and marketers if a respondent changed the device, unlike river sampling, where there is no such device tracking.
What are the two types of River Sampling?
The two types of river sampling are stratified and convenience river sampling. Stratified river sampling means drawing samples in real-time from online promotions, such as website banners, ads, pop-ups, and hyperlinks. In contrast, convenience river sampling involves placing advertisements and hyperlinks across websites without analyzing the websites' traffic. This enables marketers to gain maximum data at minimum cost.
What are some pros and cons of river sampling?
The most significant advantage of river sampling is that it makes the surveys accessible to everyone, even respondents who were not conditioned to take part in a survey. It also ensures the complete anonymity of respondents and is a relatively inexpensive way of conducting surveys. However, since these surveys ensure anonymity and there is no link to a respondent's ID, people can take the same survey repeatedly and distort any chance of accurate results.
What are some pros and cons of RDE sampling?
RDE sampling can detect different devices that respondents use, decreasing the chance of any fraud. It also provides high-quality data due to AI functionality and automated checks. However, the drawback is that RDE surveys may not exist in as diverse a set as they do in river sampling.
Online Survey Sampling Methods: Random Device Engagement & Organic Sampling
Organic Random Device Engagement Sampling Methodology
Academic whitepaper written by Dr. David Rothschild, Economist at Microsoft Research & Dr. Tobias Konitzer, C.S.O. and co-founder of PredictWise.
2015 and 2016 saw high-profile polling failures throughout the world.
In the summer of 2015, before Brexit and the 2016 US election, The New York Times asked, somewhat rhetorically:
What is the matter with political polling?
Implying that there was already a crisis of confidence in polling. Then in 2016, the United Kingdom stunned the world by voting in favor of Brexit, a referendum on the United Kingdom leaving the European Union, despite opinion polls shifting towards remain in the last few days. A few months later, despite polling showing Democratic candidate Hillary Clinton winning in enough states to win the US election, and poll aggregators confidently pointing to a Clinton victory, Republican candidate Donald Trump won a fairly comfortable Electoral College victory (but, still lost the popular vote).
While there is some nuance to the label of failure, the popular vote was forecast spectacularly well by polling aggregators, and “failure” was really a local phenomenon boiling down to a number of state-level polls in the Rust Belt (and applied to the presidential election only, and not congressional elections): the public perception was that of “failure in broad and absolute terms. As is now well known, this failure (or at least either perception of failure or partial failure) led to a reckoning with the status-quo modus operandi of polling; the whole industry faced a market-threatening question of where they were going.
Culprits were readily identified and one target was Random Digit Dialing (RDD) polling samples, the gold standard of high-quality polling in recent decades, which has undergone a massive shift in recent years. RDD response rates have decreased from 36% in 1997 to single digits in the 2010s. And, as Gelman et al. (2016) shows this non-response is coupled to political attitudes: today, traditional polls, RDD with a mix of landlines and cellphones, have a hard time reaching those with lower levels of education and lower levels of political knowledge. Thus, polls in 2016, especially the crucial state-level polls in the contiguous states of the Rust Belt, that neglected to weight on education had a huge problem. Similarly, RDD has a hard time reaching White blue-collar voters, dubbed Bowling Alone Voters, especially mobile blue-collar voters (“Truck Driver phenomenon), as a Post Mortem by Civis Analytics has pointed out. This is even harder to control with traditional analytics.
But, even more serious than its current problems: even if RDD can still work, it is doomed in next few years. Do you have a landline? Do you answer unknown (or suppressed) numbers on your cell phone? Will you have a cell phone in 10 years? Will the platform for reaching you be a phone number or a user ID? These are serious questions that further jeopardize the future of random digit dialing: by definition, it is impossible without phones!
As with all discussions around polling, it is critical to delineate two distinct things: data (or sample) collection and analytics. Data collection is how respondents are gathered. Data analytics is how the collected data is turned into market intelligence. Nothing prevents the most advanced analytics from being used on any data collection, although different analytics will provide various levels of benefit to different samples. For this paper, we will stick to data collection but refer to several previous papers exploring data analytics (Goel, Obeng and Rothschild 2015).
As is the case with all innovation, some innovation is good and scientifically sound, some innovation is snake oil, with little or no effect, and some innovation is flat-out dangerous. In this paper we shed light on three such innovations competing to replace RDD: Online (non-)probability panels, Assisted Crowdsourcing, and Random Device Engagement (RDE). All innovations come with strengths and weaknesses. But, as we spell out here, one is the clear winner: RDE, which is why RDE is at the core of our methodology.
Traditional & Online Survey Sampling Methods
Random Digit Dialing (RDD)
Random digit dialing is exactly as the name says: building a sample calling random people on the phone. The first step is to identify a cluster of phone numbers that have reasonable demographic and geographic representation. Then, start calling those numbers at random, trigger a response, and collect poll answers over the phone. The mode is confined, by definition, to a telephone, but it has recently expanded to both landline and cell phones. The mode has high coverage (in that most people have either or both a landline and cell phone), but coverage becomes harder to assess while landline penetration is dropping as cell phone penetration is rising. This makes it hard for survey researchers to map the population in either group or any individuals inclusion in either group. Response rates are oftentimes in the single digits.
Online Panels
Online panels collect responses either via a fully opt-in structure, including a signup page, or start with an RDD-telephone (and/or supplemented with cell phone) or mail recruitment. Panelists are then recruited to participate in specific surveys, for example via email invitation to the page of the panel provider. The mode is a mix of desktop, tablet, and smartphones, depending on the device of choice from which the invitation is opened. The mode has very low coverage (very few people opt-in to panels), but RDD-based panels, which start out with random methods of recruitment, have better coverage. Response rates, although generally decent from panelists, are low when one considers the low degree of opt-in to the panel. This makes them hard to compute accurately.
This has a number of advantages:
1. Panels provide repeated and connected users.
Over-time trends can be analyzed, and any custom polling built on top of baseline tracking can be guided by priors derived from data a serious innovation.
2. Online survey sampling methods like online panels are relatively cheap and fast.
Marginal polling is relatively inexpensive and can be done faster than traditional random digit dialing.
Curating panels as an online survey sampling method comes with a number of serious disadvantages:
1. You are locked into one model of data collection.
Polling firms that are locked into a specific mode of data collection will be hit with tremendous costs because the old infrastructure will have to be dismantled as technology shifts over time. And, no one can predict how long online panels will be a viable mode of data collection as web usages shifts to mobile and beyond (yes, you are reading this right: we want you to think virtual reality here). And, many companies that build their polling around this form of panel are locked into non-transferable unique identifiers of each respondent. This has some short-term benefits, but it will make it very costly when the companies need to shift data collection as technology evolves.
2. Panel fatigue
A myriad of research has documented that repeated participation in polls of panelists can lead to panel fatigue, resulting in non-response error or measurement error (Porter, Whitcomb and Weitzer 2004; Kasprzyk 2005). The applied scenario: respondents might be eager to fill out surveys correctly and with care, but this willingness declines the more respondents are invited to participate in surveys, especially if respondents are at risk to lose panel status. Instead of providing meaningful answers, respondents then click random answer options, or gravitate toward "Don't know".
3. Panel effects/Panel conditioning
Slightly different from panel fatigue are panel effects, or panel conditioning (Sturgis, Allum and Brunton-Smith 2009; Halpern-Manners, Warren and Torche 2017). Even if panels recruit a sample that looks like the perfect cross-section of the desired target population at the time of recruitment, the demand to answer political surveys turns these initially representative panelists into a bunch of very politically aware citizens. Panel conditioning has plagued a number of panels or panel-like setups. In the worst-case scenario, all panelists will have acquired a base degree of political sophistication as a consequence of being professional political survey takers. In that case, even the most advanced bias correction algorithms will fail because of sharp separation: Among the panelists, no one (read: zero) who mimics the stratum with low levels of political sophistication is left.
4. Mix of web and mobile not clean
Web panels tend to engage respondents either desktop, or on their mobile devices, but the infrastructure may, or may not, be very adaptive. Either way, the users are engaging in different experiences conditional on the device of engagement, which is hard to control for.
5. Non-Organic
In panels, respondents are not engaged in their natural (read: organic) environment (Zaller et al. 1992). Instead, an alternative digital environment is created, with the potential of introducing measurement error. As respondents are taken out of their normal routine, thought processes can deviate from those in more natural environments, leading to artificial considerations that can unduly influence item response.
Online panels have the ability to track public sentiment over time more easily than RDD, and are able to leverage the longitudinal panel structure of the data to parse out true swings from artificial movements. In addition, clients of custom polls can be guided by a plethora of prior baseline data when writing the poll. But, reliance on online survey sampling methods of data collection and dangers of panel fatigue and panel conditioning mean that insights can be seriously biased, especially if the panel exists for a longer period of time (and panels, as a class, exist for a longer period of time) and it is getting harder to recruit a fresh replacement sample.
Assisted Crowdsourcing
Assisted crowdsourcing polling relies on social networks with massive penetration, and data on their users, to supply respondents (read Facebook: while it can be done on other display or search ad platforms, the massive penetration/coverage and availability of background demographic data mean that Facebook is really one of the few alternatives).
First, the researcher creates a set of demographic quotas (i.e., the number of respondents they want with any combination of demographics). She then submits these quotas to a social media platform, along with an ad to invite respondents to participate in the survey. The social network then serves this content to a targeted group of users, and the polling firm surveys respondents who click on the ad and go to the survey site. The mode is mainly desktop, but could be tablet or mobile as well. This method has very high coverage, but low response rates.
There are some advantages with this sampling method:
1. Speed and Targeting
The main advantage here is that due to the penetration and reach of Facebook, polling can be done at granular areas (think state legislative districts), at a somewhat cheaper cost (by our estimates, respondents will run at about $5). Thus, a polling firm engaging in assisted crowdsourcing could sell a poll of N 1,000 for about $8,000-$10,000, slightly cheaper than traditional polls (but with a similar cost to online panels), and, due to Facebooks reach, faster. In summary, good depth, speed, and relatively good costs.
2. Organic Sample
Facebook is an organic location for getting opinions. Instead of curating professional survey takers who answer many political polls akin to a (side-)job, assisted crowdsourcing reaches respondents where they spend time organically. That is to say, people live on Facebook, get their information on Facebook, share their thoughts on Facebook; assisted crowdsourcing gathers opinions in that natural environment.
There are BIGGER disadvantages:
1. Quota Sampling is bad
Quota sampling has long been shunned by high-quality polls, and for good reasons: The debacle in the 1948 election laid bare the dangers in quota sampling (i.e., Dewey did not beat Truman). If respondents are “recruited to fill demographic buckets, pollsters are going to recruit respondents in that bucket who are easiest to reach. You need to recruit 10 non-college educated Whites? Great, you have interacted with representatives of that demographic bucket in the past, why not simply recruit these folks? While this is done in practice, hitting the same respondents over and over again is problematic. More importantly, the ability to reach someone within a bucket is likely correlated with the respondents level of political engagement, partisan affiliation, and political knowledge: the same things you are trying to measure. Specifically, respondents of certain demographic strata who are easy to reach have abnormally high levels of political engagement, knowledge, etc., leading to a sizable bias that cannot easily be corrected.
2. Quota sampling on social networks is worse
If you are dealing with social networks, the quota sampling problem discussed above gets much worse. Facebook algorithms are designed to expose the cheapest respondent to the ad, i.e. the respondent that is most likely to maximize click-through rates (see for example this discussion about Facebook targeting algorithms in this recent PNAS letter (Eckles, Gordon and Johnson 2018)). Hence, it makes sense to show ads to participate in a political survey, especially those that have a political cue, to users who are more likely to click on political content for example users who declare a self-reported ideology as part of their profile, or like a lot of political content.
If polling firms relying on assisted crowdsourcing target, say, non-college educated Whites, chances are that those non-college educated Whites who are exposed to the ad because of their high likelihood to click on political content exhibit unusually high levels of political engagement. To make matters worse, the characteristics most predictive of that non-representativeness, behavioral metrics from Facebook such as Likes of political content, are not available to polling firms for bias correction. And, in expectation, Facebook's machine-learning algorithms get better at predicting who clicks on ads to participate in political polls, and who does not, over time. This means that (a) biases exacerbate the longer polling firms recruit respondents on Facebook, and (b) the number of fresh respondents diminishes, in effect leading to a panel structure bringing with it concerns of measurement error due to panel fatigue, or panel conditioning effects, meaning a change in underlying attitudes as a direct consequence of membership in a panel-like structure.
3. Assisted crowdsourcing is at the mercy of social networks
Simply, any survey tool on a social network is reliant on the legal framework surrounding social networks with high penetration (and, there are really only two or three to speak of). Much like online panels, assisted crowdsourcing lacks agility with technology and adaptability to new audiences. Should any preemptive legislative strike result in the social networks withdrawal from the political ad market (or a dramatic shift in costs or types of exposure), a possible scenario amidst the recent turmoil surrounding the data breach leveraged by the now-defunct right-wing analytics firm Cambridge Analytica, the respondent market and methodology fine-tuned to the idiosyncrasies of respondents drawn from the social network in question, can become obsolete in a matter of minutes.
Polling companies relying on Assisted crowdsourcing have the ability to poll every political race from presidential elections to state legislative elections, and that is commendable. But, biases introduced by quota sampling, exacerbated by fine-tuned targeting algorithms of social networks, meaning that severe and uncorrectable sample bias can lead to serious polling error. In addition, the nature and extent of respondent supply are completely dependent on a legal framework polling firms have no influence over.
Random Device Engagement
Many of the tenants of RDD are commendable: calling respondents in their homes means that respondents are picked up in an organic location for getting opinions. Pollsters reach respondents where they spend time organically. That is to say, people engage in their quotidian tasks at home, get information at home, and interact with friends and family. In short, RDD gathers opinions in that natural environment. Can we fix what is broken with RDD while maintaining its strengths?
Let us introduce Random Device Engagement (RDE); it is the natural successor of RDD, in terms of orthography, philosophy, and quality.
Random device engagement (RDE) polling relies on advertising networks, or other portals on devices, to engage random people where they are. One of the most common versions of this is within advertising modules on smartphones, but it can easily be placed in gaming, virtual reality, etc. Survey respondents are asked to participate in a poll in exchange for an incentive token that stays true to the philosophy of the app in which they are engaged: For example, respondents contacted via the popular mobile gaming App Harry Potter: Hogwarts Mystery can be reimbursed for survey participation with energy points, a crucial currency of the game. Direct monetary incentives are also possible, such as the chance to win an Amazon gift certificate.
The key here is that by being able to monitor the unique identifier of the device world ad IDs survey firms can prevent fraud originating from SUMAs (single users, multiple accounts). And, RDE samples are both random and organic. This is the natural successor to random digit dialing, which aims to randomly engage with landline (and now cell) phones. In many ways, it is just making RDD generic for the future: random, device (rather than phone), engagement (rather than dialing). It addresses RDDs greatest problem: technology is always changing. It solves for this by targeting a respondent's unique ID that can be tracked across changing devices, as the future of phones is uncertain. In addition, RDE brings a plethora of telemetry or para data to the table that is amenable to bias correction, from location history to application usage.
This method has a number of advantages:
1. Fast
RDE can be extremely fast. RDD takes days (and weeks in some cases). Using social networks (assisted crowdsourcing) can be done a little faster, but still lacks speed compared to RDE. Using online panels is comparable in speed, if you pay for extra respondents from a merged panel (online panels will charge extra to get respondents from other panels to increase speed).
2. Cost-effective
RDE is extremely inexpensive compared with other sampling 12 options. The major RDE providers, like Pollfish, Dalia or Tap Research, charge 10% the cost of RDD, 20% the cost of using assisted crowdsourcing, and 25% the cost of online panels.
3. Coverage is good and growing
Accuracy is good because coverage is good. The major RDE providers mentioned easily reach 5,000,000 unique respondents, in the US market alone. And, while RDE is still behind RDD in coverage at this time, it will reach parity soon. Coverage is similar to social media-based assisted crowdsource polling and much better than with online panels. Online panels have a very small footprint, which also affects their ability to get depth in population.
4. Response rate is solid
Pollfish reports a reasonable response rate (much higher than RDD), conditional on being targeted for a poll (to completion of the survey, that is). Online panels have low sign-up rates and high drop out but do not post comparable response rates. Social media-based polling, in assisted crowdsourcing, is reliant on ads that suffer from a very low click-through.
5. Flexible
RDE is meant to be flexible with the growth of devices. It should provide a seamless experience across device types. RDD is stuck with telephones, by definition. And, RDD is subject to interviewer effects (albeit to a smaller extent than in-person surveys), meaning that tone of voice can influence considerations of the respondent, or trigger undesired interviewer respondent interactions, ultimately introducing measurement error. RDE, with its streamlined experience, is not subject to this kind of error. (Tucker 1983; West and Blom 2017)
6. Telemetry data
RDE is able to supplement collected attitudinal data with a rich array of para or telemetry data. As we know, people who answer surveys are fundamentally different than people who do not. As the progressive analytics shop, CIVIS has argued recently, a battery of nearly 30 additional demographic, attitudinal, and lifestyle questions that get at notions of social trust and cosmopolitanism is necessary to be able to weight and correct for all the ways in which survey respondents are unusual. As Konitzer, Eckman and Rothschild (2016) argue, telemetry data is a much more cost-effective (and unobtrusive) way to collect these variables. Home and work location, commuting or mobility patterns or the political makeup of one's neighborhood or social network, derived from satellite-based (read: extremely accurate) longitudinal location-coordinate data predict demographic variables well, such as race and income. And, applications on the device can more accurately describe political traits prone to erroneous self-report, such as frequency of political discussion, political engagement or knowledge.
7. RDE will get stronger in the future
Penetration of devices will further increase in the future, increasing reach of RDE in the US, and making RDE the only viable alternatives in less developed markets. Take Africa: the smartphone penetration rate is projected to grow at 52.9% year-on-year. Currently, there are 293 million smartphone users across the continent, meaning that taking into account current growth rates, there will be 929.9 million smartphones by the year 2021 in Africa. But the rosy future for RDE is not just about penetration. Advances in bridging Ad IDs with other known identifiers in the American market, such as voter file IDs, Experian Gold IDs, etc., mean that individual targeting based on financial history or credit card spending patterns will be possible. And, RDE will be able to adopt list-based polling, in which political survey firms poll directly from the voter file, large-scale administrative data detailing the turnout and registration history of 250,000,000 Americans.
8. River sampling is different, as devices are unknown
River sampling can either mean banner-ad based polling or engagement with respondents via legacy websites or similar places RDE recruits from. In contrast to RDE, devices are unknown to river samplers: River sampling usually does not have access to the Ad ID, introducing two huge disadvantages: River samples have no way to address SUMA it is possible for fraudsters to engage with the same poll twice to increase chances to win the price for participation, especially if it comes in the form of financial incentives. And, any degree of demographic/geographic (not to mention individual) targeting is virtually impossible. In addition, banner ads themselves, similar to social-media ads, suffer from disastrous response rates. Good RDE polling is done with the cooperation of the publisher, providing a native experience, while banners ads are pushed through the ad-network. This degraded user experience depresses response rates and can introduce serious measurement error.
Second, ad-networks optimize their delivery in a way that fights against the random sample. The users are chosen because they are more likely to respond, due to unobserved variables (at least to the survey researcher), that are correlated with how they will respond. As this underlying data is never shared, it is impossible to correct for by the survey researcher.
This method has some disadvantages:
Just like every other modern online survey sampling method (RDD, assisted crowdsourcing, online panels), RDE relies on non-probability sampling. There is no sample method (anymore) that has perfect coverage and known probabilities for any respondent. This is one of the reasons we have developed analytics to overcome known biases. And, RDE has bias that we understand and can overcome, and additional data points that add to the power of correcting bias, such as telemetry data that is not available to RDD. While RDD has shifting and shrinking coverage, online panels suffer from panel fatigue and panel conditioning, and assisted crowdsourcing has survey bias introduced by efficient but to the polling firm nontransparent targeting algorithms that cannot be addressed, RDE is our method of choice, and the future, in the ever-changing market of polling.
Examples of RDE
Here we review work published in both Goel, Obeng and Rothschild (2015) and Konitzer, Corbett-Davies and Rothschild (N.d.) to showcase how effective RDE samples can be. And, add examples from the 2017-2018 special congressional elections.
Example 1:
(Goel, Obeng and Rothschild 2015) shows how RDE, through Pollfish, is able to closely match gold-standard polling such as the General Social Survey. This gold-standard uses yet another method: house-calls. This is unaffordable for most research, so we have left it off of this paper, but it provides a useful benchmark.
Example 2:
(Konitzer, Corbett-Davies and Rothschild N.d.) shows how RDE, utilizing the Pollfish platform, is able to closely match RDD polling in the 2016 election (actually doing slightly better). This is an example of using RDE samples with an analytic method call Dynamic MRP. The analytics methods are detailed in their paper.
When (Konitzer, Corbett-Davies and Rothschild N.d.) quantifies their state-by-state errors, they show that their predictions based on a single poll are not significantly worse than the predictions from poll aggregators. They compare their state-by-state estimates against the actual outcome. Compared to poll aggregator Huffington Post Pollster, their Root Mean Squared Error (RMSE) is only slightly higher: 4.24 percentage points vs. 3.62 percentage points (for 50 states excluding DC).
When they focus on the 15 closest states, predictive accuracy is even higher. The RMSE is 2.89 percentage points, compared to 2.57 percentage points of Huffington Post Pollster. Overall, besides binary accuracy the RDE-based polling predictions also have a low error in the precise percentage value.
This is illustrated in Figure 1.
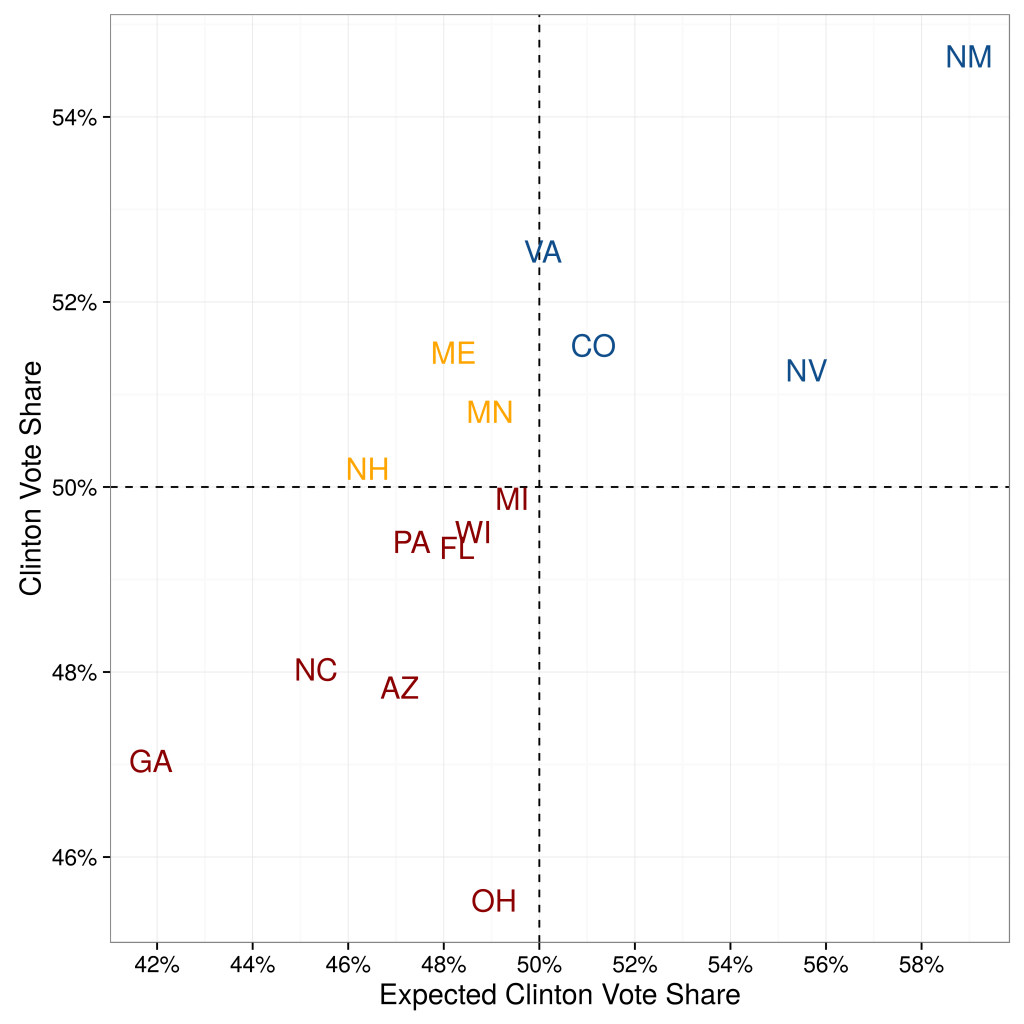
Not only are RDE-based polling state-by-state estimations fairly accurate, they also add meaningful signal to the poll aggregations. The left panel of Figure 2 displays the correlation between state-by-state errors of our predictions and the state-by-state errors of Huffington Post Pollster, and the right panel compares the distribution of errors across their approach and Huffington Post Pollster. At the very least, using RDE has significant potential to increase the quality of aggregators, as we discuss more below.
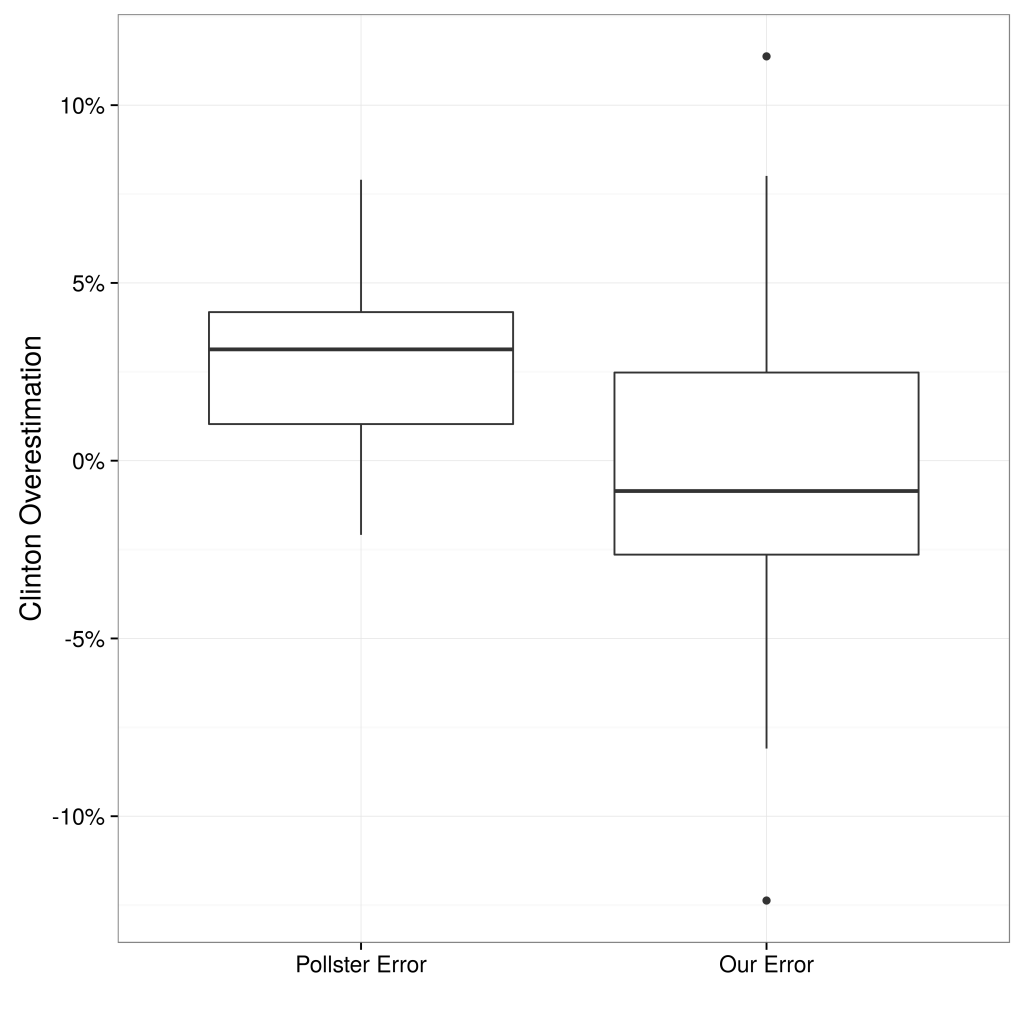
Example 3:
During the course of 2017 and 2018 polling firms have employed all three new methods in predicting Congressional election outcomes: RDE comes out way above the other two.
In this paper we outlined four methods of data collection for surveys. The first method, Random Digit Dialing (RDD), is the traditional method, working fine, but it is doomed in the next few years. Thus, the paper is really about which of the new online survey sampling methods will replace it: online panels, Assisted Crowdsourcing, or Random Device Engagment (RDE). We believe strongly that RDE is the future.