How to Conduct Exploratory Research for Your Early Research Needs
How to Conduct Exploratory Research for Your Early Research Needs
Exploratory research is one of the main types of general and survey research. It works to investigate an issue, occurrence or phenomenon that is not clearly defined.
The most preliminary form of research, exploratory precedes descriptive research, another early survey research campaign.
As such, market researchers and virtually all other researchers need to incorporate exploratory research in their market research campaigns, as they would be remiss to not fully comprehend a problem before probing further into it or attempting to fix it.
This article examines exploratory research, what makes it up, how to conduct it, how it differs from the other main forms of research and the kinds of surveys to use to carry out this research.
Defining Exploratory Research
Exploratory research is defined as an initial form of research that studies a hypothetical or theoretical idea, that is, one which has not yet been fully developed, let alone proven.
As such, this research begins with a researcher’s idea about something within their sphere of study; for example, they’ve noticed interest around their brand has fallen in a particular quarter.
The researcher will thus use exploratory research to gain a better understanding of this unexplored idea, prove that it exists in significant ways/amounts and study other issues surrounding it.
The issues and other details that they discover can be carried over as the focus for future research campaigns, namely descriptive research, as the next logical type of research.
Exploratory research is defined as a research used to investigate a problem which is not clearly defined. It is conducted to have a better understanding of the existing problem, but will not provide conclusive results.
Also called grounded theory approach or interpretive research, exploratory research helps answer questions like the “what,” “why” and “how.”
The Key Aspects of Exploratory Research
Now that we have established the core meaning and function of exploratory research, it is critical to understand its makeup. This form of research has various qualities researchers ought to look into, to better understand its characteristics.
The following enumerates the key features of this research:
- The initial form of research around a particular subject of study.
- Lays the groundwork about a study for future research.
- Investigates an issue that is not fully defined.
- Gathers information that can be explored in more depth in descriptive research.
- Exists in two forms: Via a new topic or via a new angle
- A new topic is usually unexpected and provides startling findings.
- A new angle arises from different ways of looking at things, either from a theoretical perspective or a new way of measuring something.
- Enables a researcher to answer foundational questions such as: What is the problem? What is the purpose of the research? What topics should be studied?
- Exploratory research uses unstructured studies.
- Involves forming a few theories which can support its findings to make it easier for the researcher to assess them.
- Typically involves yielding qualitative research.
- Can produce quantitative research that can be used to generalize larger samples in certain cases, such as through the use of experiments and quantitative surveys.
Why Your Business Needs Exploratory Research
Businesses need exploratory research for a variety of reasons. Firstly, this research method uncovers details and facts about a murky subject — the kinds that a business hasn’t previously known about.
It sets the foundation for understanding a problem, occurrence or phenomenon by finding its basic properties. This ensures that a business finds the right information (such as the variables) which can be further studied in descriptive, correlational and experimental research.
Although it rarely provides enough insights to make conclusive business decisions, it forms the basis of a research issue on which businesses can set up objectives and requirements for continual studies.
After all, a company cannot conduct further research around a topic, without assuredly knowing about its existence and certain characteristics that warrant further exploration.
With exploratory research, businesses extract:
- The groundwork for other types of research
- Key facts about matters critical to their business
- Opinions from their target market
- The existence of specific market segments (via market segmentation)
- An understanding what is worth pursuing for survey studies and other research
Exploratory Research Survey Examples
- The qualitative survey
- Helps answer the what, why and how with open-ended questions.
- Extracts key high-level information in depth.
- The cross-sectional survey
- Studies a particular population at one particular point in time.
- Can help form a hypothesis of for example, shopping behavior during a seasonal campaign.
- The customer experience (CX) survey
- Finds foundational insights on customers’ CX.
- Finds glitches and other issues in a customer journey that a business was not aware of.
- The employee feedback survey
- Finds unknown employee sentiment around various areas of business.
- Useful during mergers, acquisitions, growth spurts or simple quarterly reviews.
- The business survey
- For general inquiries, understanding the high level details.
- Although it can be used for other forms of research, this survey can help identify a problem.
How Exploratory Research Differs from Correlational, Exploratory and Experimental Research
Exploratory research differs significantly from the other main types of research methods such as correlational research and experimental research.
However, exploratory research is often conflated with descriptive research. Although both forms of research are conducted in the early stages of the entire research process, they are not the same, as they bear key differing qualities.
Exploratory research provides the foundation, hypothesis or discovery about a problem the researcher suspects is present. Thus, it is the very first form of research required to conduct over an unstudied or unknown topic.
Descriptive research, on the other hand, is predicated on describing something already established, discovered or suspected in exploratory research. Thus descriptive research follows exploratory research in the overall research process. It describes characteristics, functions, occurrences, frequencies and other required key facts before the researcher moves to correlational or experimental research.
Descriptive research, as opposed to exploratory research, is conclusive. It is predominantly quantitative and fixed on creating statistics. It is also rigid and structured, while exploratory research is flexible and unstructured.
Correlational research differs from exploratory research in that it is one of the latter forms or research, whereas exploratory is the most preliminary kind.
It processes well-established information that exploratory and descriptive research have found. Its primary function is to uncover relationships among variables to see how one may affect another or others.
The results of correlational research can be used to make predictions of future events from present insights.
Experimental research contrasts with exploratory research, in that it is also one of the latter forms of research, if not the very final. Unlike exploratory research, experimental research is far less observational or passive. Rather, as its name hints at, it is highly experimental, as it uses a scientific approach on two sets of variables.
Using a scientific research design, it forms experiments to find cause-and-effect relationships between defined variables. Also called hypothesis testing or a deductive research method, itis conducted in a controlled environment so that variables can be measured, calculated and then compared. .
Experimental research involves manipulating variables to come to a conclusion or finding. It helps find conclusions to an original subject of research or answers to a previously discovered problem in exploratory research.
The Advantages and Disadvantages of Exploratory Research
Exploratory research offers several benefits for researchers and businesses. However, as with all other research methods, there are a few setbacks to this type of research.
The Advantages
- Enables further research on an established issue.
- Unstructured, allowing the researcher to be flexible in their study.
- A low-cost form of research.
- Allows researchers to decide whether a topic is worth studying further or not.
- Can save valuable time, money and resources, if a discovered topic is deemed unnecessary for further research.
- Helps researchers in the early stages of discovering the cause of a problem.
- Points researchers to an objective or signals researchers to avoid some.
The Disadvantages
- Qualitative data, the main kind of data derived from exploratory research, can be easy to misinterpret when generalizing a population.
- Although it steers further research, it is inconclusive.
- Although it can be used in different survey sampling methods, it usually involves using a smaller sampling pool, making it inept at generalizing populations.
- When collected via secondary sources, the data may be outdated and thus not fully accurate with the present.
How to Conduct Exploratory Research
This form of research is known to be unstructured, so there are no hard and fast rules on how to conduct it, as long as it adheres to its key aspects. The following explains the basic universal approach for conducting exploratory research.
- Identify the problem: The core of the research, this may take a few brainstorming sessions.
- Accrue secondary research that relates to your suspected problem, issue or phenomenon.
- Conduct a basic survey to see if there is any truth to your identified issue.
- Create the hypothesis: If there are no prior studies or the problem is not fully under control or has a workaround form a hypothesis based on research from the previous step.
- Conduct further research: Continue researching to see if there is any truth to the existence of the problem and its possible solution/hypothesis.
- Conduct surveys, focus groups, interviews and more secondary research.
- Decide whether the subject at large and its hypothesis are worth delving into further in descriptive research.
Excelling in Exploratory Research
Exploratory research forms the building blocks of an overall research project, as it constructs the groundwork of a research subject that will later be explored (in descriptive research), tested (in correlational research) and manipulated in (experimental research).
As a researcher, perhaps there will be times that you won’t need to conduct much exploratory research. This is true in cases where you have some familiarity with a subject or are certain of its existence in your business, in which case, you can proceed to descriptive or experimental research.
In any case, you need to be fully aware of a problem or other phenomenon before conducting any further research on it. This is where exploratory research excels.
In order to be skillful in exploratory research, you need to turn to several resources, such as secondary research, case studies, literature within your industry/niche and finally, surveys. Although surveys ask for specifics, they too are invaluable for conducting exploratory research, as they help uncover the what, giving credence to any suspicions you may have had prior to endeavoring in research.
As such, using a potent online survey platform will assist, guide and launch the key tool in your exploratory research.
Frequently asked questions
What is exploratory research?
Exploratory research is conducted on ideas that are not fully developed. Researchers usually start brainstorming an idea that lies within their specialization and develop it into a broader concept.
What are the key features of exploratory research?
The key features of exploratory research include the initial research on a specific topic, laying the foundation of the research topic, investigating the issue, and collecting information that supports descriptive research.
Why is exploratory research important for businesses?
Exploratory research is vital for businesses, as it helps them understand the root causes of their problems and find ways to solve them. As a result, businesses find the correct information and access appropriate resources to develop the chosen idea. It can also help enterprises to set up objectives for continual development studies.
What kind of surveys can you make with exploratory research?
Exploratory research can help you conduct:
Qualitative surveys – brilliant for gathering in-depth information and open-ended questions
Cross-sectional surveys – suitable for studying a sample size of a particular demographic at a time
Employee feedback surveys – help understand how employees feel about the workplace and their work
What is the difference between exploratory research and experimental research?
Exploratory research takes an idea and develops it further. But experimental research is far less observational as it uses a scientific approach to test two sets of variables.
The Complete Guide to Mastering the 6 Most Critical Types of Research for Any Research Endeavor
The Complete Guide to Mastering the 6 Most Critical Types of Research for Any Research Endeavor
Understanding the six most critical types of research is an absolute must for market researchers and general researchers alike.
The world of research is ever-expanding as new technologies evolve, new techniques for obtaining data arise and more secondary sources become available to the public.
However, the six chief types of research remain as the foremost processes for conducting investigations. They refer to specific types of research which include more than merely using a method of study.
This guide explains the six prominent types of research, when to use each, how they benefit business and more.
Defining the Major Types of Research
For the purpose of general research, a major type of research does not refer to conducting studies on a designated topic of choice (for example, sales research).
So what defines a “major” type of research?
When categorizing research into several key varieties, a “type of research'' refers to a particular form of research that can examine virtually any topic and its variables, thorough particular means and approaches. These approaches involve using distinct components such as methods, processes and frequencies particular to one kind of research.
These components form the core of the research type, making it feasible to differentiate from others. Each variety of research is also bound by a unique purpose. This purpose is not thematic, as it can be applied to all kinds of subjects of study.
Despite operating through different approaches and methods, some forms of research share several features, including the purpose of the study/ the kind of results it seeks to some extent.
The Need to Understand the Different Types of Research
Whether you operate under a B2C or a B2B business, either as a business owner or market researcher, you ought to verse yourself in the different types of research. This includes being able to distinguish between them and not confusing one for another.
Before you tackle any area of concern to investigate for your research needs, you need to assure you’re setting your research project up for success. In order to form an effective research campaign, you’ll need to be methodical.
This means you’ll need to tend to several concerns to build a successful campaign. This involves organizing your topic of study and inquiries into a particular variety of research.
Doing so will ensure you apply the correct market research techniques and methods, the kinds that best suit the inquiries and needs of your topic of research, thus, best tending to your concerns.
When you use the correct type of research for your study, you’ll be able to understand it more thoroughly and thereby find more fitting changes and solutions. This is especially true when your area of study is a problem you would like to minimize or reverse.
Using the correct form of research will also ensure that you are measuring and observing the correct elements and by way of a frequency best suited towards your research issue.
Moreover, when you employ the proper type of research, it is far less likely to come upon errors and gaps that require answers. Thus, there is less of a need to start again or switch to a different type of research.
All of these areas of importance would be impossible to fulfill if you do not become familiar with them and are not able to tell them apart.
The following explains the six most critical types of research.
Exploratory Research
What it is: Used to reveal facts and details around a topic with little to no research, exploratory research forms the foundation of the research process. It identifies a topic, be it an issue or a phenomenon with scant details and seeks to find its basic properties.
As such, it finds the correct variables the researcher needs in order to begin the study, understand its basic elements and form a hypothesis. The key issue at hand, its variables and its hypothesis are used for further research.
Essentially, this kind of research forms the premise of a research campaign, assuring that the variables and other components are indeed what the researcher needs to study in the next steps (other types of research).
Stage in the research process: The very first
Conclusive? No
How it benefits a business: Before a business can explore an issue in-depth, it needs to decide on a specific topic, the existing problem within the topic and its key variables. This ensures the business is equipped to enter the next research stage (type) and that it does not have any extraneous variables or concerns that do not contribute to solving the problem.
Descriptive Research
What it is: This type of research is premised on describing a phenomenon, behavior or problem discovered in an earlier stage of research, usually in exploratory research, although it can also be focused around that which was discovered in explanatory research.
Descriptive research describes the nuances of a population, a variable or occurrence that a researcher requires further study on. Its objective centers on finding previously unknown facts or extracting more details on facets with fewer details.
It focuses on the what, how, when and where of a study rather than on the why.
Stage in the research process: The early portion of the middle stage
Conclusive? Yes
How it benefits a business: It is crucial for a business to understand a phenomenon and its variables in a full or close-to-full context. This type of research helps a business do just that, as it finds all the key details about a phenomenon that a business may not have known about before conducting the research.
What’s more is that, as a primarily quantitative form of research, it is apt for creating statistics. Being statistically-oriented allows this form of research to be conclusive, although it is considered to be in the early mid-stages of an entire research project.
These statistics are not simply key for internal resource purposes, but they provide a differentiating ingredient for your content. A strong content marketing strategy relies on putting out original insights; the data you derive from descriptive research is as original as it gets. This can be accomplished when you opt for a primary method (such as survey research).
Explanatory Research
What it is: Explanatory research is based on research that explains the already established aspects in a research campaign. It fills in the gaps and connects the dots from exploratory and descriptive research.
This type of research is unique in that it can be conducted either prior to or after descriptive research. As such, it rests in the early to mid-stages of the overall research process.
Like descriptive research, it works to shine a light on the various details that make up a research subject of study. However, contrary to descriptive research, it does not simply seek to describe, but rather to explain.
Thus, this research category falls under qualitative research. It helps find the why of a problem or phenomenon. It is not conclusive.
Stage in the research process: Early to mid-stages (can be performed before or after descriptive research, depending on a business’s needs).
Conclusive? No
How it benefits a business: It benefits a business in that it seeks to go beyond describing a subject of study. Rather, it plunges into a subject in greater depth, finding the kinds of insights that descriptive research cannot.
Additionally, it is flexible. It can be conducted following exploratory research and either before or after descriptive research, the only research of its kind to offer this benefit.
This research involves studying an important aspect that is studied in the later stages of the entire process, that of cause and effect. Explanatory research studies cause and effect relationships so as to explain their scope and nature, a critical precursor for correlational and causal research.
Correlational Research
What it is: Correlational research is a study into the relationship between two variables. Inspecting precisely two variables, this type of research seeks to discover and render the relationship between variables suspected of relating in some way.
This research seeks to make sense out of the variables identified in earlier stages of research. Although correlational research is not sufficient to conclude on cause and effect relationships, it is necessary to conduct to find whether a relationship between variables exists to begin with.
An observational form of research, it is non-experimental; there is no controlling or manipulation of the variables involved.
The relationship between the variables can be either positive, negative or zero (nonexistent).
Stage in the research process: Middle stage
Conclusive? No
How it benefits a business: Being able to determine if there is a positive, negative or zero correlation between two variables allows researchers to know how to move on to the next step: finding a cause and effect relationship between the variables.
A zero correlation informs a business that there's no need to further study the relationship between two particular variables, saving the business money and time. A negative or positive correlation dictates that further research is needed to discover whether there is cause and effect relationship.
Either way, the results derived from this type of research are highly influential on the next steps a business decides to take in their research process: whether to end it, continue and how.
Above all, it reveals how two variables relate to one other, giving a business a clearer picture of the environment they operate within, whether the variables concern sales figures, impressions or something more abstract like customer loyalty.
Causal Research
What it is: Causal research is founded on the undertaking of determining cause and effect relationships. As such, it involves conducting experiments and testing markets in a controlled setting. It is more scientific than any of the previous types of research.
This kind of research uses the findings from correlational and explanatory research in an attempt to unearth causal relationships. Since correlation does not equal causation, causal research studies whether the variables with a negative or positive correlation have any effect on the other variable(s) in the study.
Causal research has two objectives: finding which variable forms the cause and which makes up the effect, and understanding the relationship of the causal variables after the effect occurs.
Stage in the research process: Late-final stage
Conclusive? Yes
How it benefits a business: Often the final form of research, causal research is critical to complete the entire process. It involves conducting both secondary and primary research, the latter of which is experimental.
As such, this research type does not only observe, rather it investigates the variables themselves, manipulating them and controlling them as needed. This is crucial for a business in that it not only analyzes, but proves the existence of a causal relationship, along with how the effect manifests.
Thus, this research is not only conclusive, as it finds the most important result that a business or market researcher seeks: a proven answer to their hypothesis. This allows researchers to close off the research process, or conduct further experimental research if they so choose.
Experimental Research
What it is: Experimental research vigorously follows a scientific research design. It is entirely scientific, more so than causal research, as it nearly, if not fully implements the scientific method towards finding a solution.
The final stage of the research process, this kind of research uses all the information from the previous stages to conduct an experiment to test a hypothesis. It can also follow causal research; causal research itself is a kind of experimental research.
Researchers can conduct further experiments on the variables they found causal relationships for, in that they can test how to reverse an unwanted correlation, or minimize it to some degree. Or, further experiments can show a business how to reap more benefits from a desired correlation.
Stage in the research process: Final stage
Conclusive? Yes
How it benefits a business: Experimental research proves or disproves a hypothesis; as such, it is the final stage in the research process. It is the most scientific kind, leaving little to no room for errors, intuition or bias.
It can be used to accommodate causal research, digging further into a discovered cause and effect relationship. This is especially important for a business, as while it is critical to know whether a causal relationship exists, understanding how to move forward with this knowledge is of the essence.
Experimental research allows brands to test discovered causal relationships further, finding much-needed solutions. For example, a brand may want to learn how to reduce an unwanted correlation or to increase a needed correlation. Moreover, conducting further experiments can show brands how to gain a desired causal relationship sooner.
Complementing Your Research
In summary, there are six major types of research. A market researcher must consider these carefully before setting up their market research campaign. In order to build a comprehensive and effective study, you need to be able to organize your research.
To begin this endeavor, you need to classify your research topic(s) under a particular campaign, such as advertising, for example. Following this, you need to create a smooth and educated process. Thus, you need to follow the research process by way of the 6 dominant forms of research that this guide explains.
Doing so will ensure you conduct a comprehensive research campaign, one that leaves little to discover, except for possible future events, In order to complement your research, you need to conduct effective surveys for research campaigns. These allow you to understand your target market or target population. Even in experimental research, conducting surveys helps fill in the cracks and find answers to the unknown. Understanding your respondents, i.e., customers is paramount for a business. The proper online survey tool does not solely compliment a business or research endeavor, it completes it.
Frequently asked questions
Why is it important for marketers to understand different types of research?
Your business may cater to unique customer segments, including people of different ages and interests. This is why it's important for any business owner and marketer to use the right kind of research methodology for their research campaign. The correct type of research enables you to understand the data more thoroughly and find more fitting changes and solutions.
What are the 6 most critical types of research?
The six critical types of research include exploratory research, descriptive research, explanatory research, correlational research, and causal research.
How do you get accurate information for your research campaigns?
To get accurate information for your research campaigns, it is essential that you make effective survey questions that enable you to understand your customers on a deeper level. Even if your research is experimental, it is necessary to complement it by conducting surveys to help fill in the gaps.
What is conclusive research and which research methods are conclusive?
Conclusive research tends to be quantitative in nature and helps marketers reach a decision. Experimental research, causal research, and descriptive research are all conclusive as they require data-sets analyzed to help reach a conclusion.
Why is exploratory research the first step in a research campaign?
Before a business can conduct an in-depth study on a particular topic or its customers, it is important first to understand the existing problem and how the research can help fix it. This information can form the trajectory for the business to enter the next research stage and make it clear what kind of research to conduct next.
Market Research Survey: The Complete Guide
Market Research Survey: The Complete Guide
Market research is a broad category of research, encompassing a concerted effort to collect information about an industry and its target market.
This process involves gathering primary (self-conducted) and secondary (information already researched and made available) sources, to fully assess how a business will fare within a particular market and audience.
A market research survey is typically a source of primary information that businesses can use as part of their market research campaigns. It can also exist as a secondary source, in which case, its studies and results are published online or in a print publication.
This article will take a close look at the market research survey, so that you can use it to the optimum benefit for your business.
What Can you Achieve with Market Research?
A market research survey, as its name entails, is used for research purposes. Before we dive into all the aspects of this survey, it is apt to learn how you can use market research to your full advantage.
Market research is critical for a variety of purposes, including marketing, advertising, and branding campaigns.
Aside from providing data-based support for these macro purposes, market research gains you invaluable insight into particular markets. For example, you may consider running a research campaign for the retail market. Market research will help you gather all the relevant information pertaining to this specific market.
Aside from retail, you can conduct market research in a number of verticals, including ecommerce, technology, real estate and many others.
There are plenty of other applications for market research. Here are some of the ways to use market research to your advantage:
- Observe data to prepare for challenges in advance
- Gauge the demand for your product or service
- Learn key market trends and staples
- Discover how your competitors are winning or losing
- Uncover your target market’s desires, preferences, aversions and thoughts
The final point is remarkably crucial for market research and for generally keeping your business afloat. And so, we’ll now dig deep into the market research survey, as this tool is especially useful for this purpose.
Defining a Market Research Survey
This tool is the most commonly used market research method — and for good reason. A market research survey allows you to gather data on your target market. Moreover, it allows businesses to do so by accessing any insights they need, as long as they form corresponding questions to their investigation.
Surveys have a far-reaching history, as they date back to ancient civilizations such as Greece and Rome. There was a surge in survey use in 1930s America, in which the government sought to understand the economic and social state of the nation.
Surveys have taken up a variety of forms, including analog forms, such as paper and mail-in formats.
Telephone surveys were the medium of choice for survey research during the 1960s-90s. But, as technological advancements would have it, those have declined in usefulness as well.
In the present day, surveys are conducted online, particularly through the use of designated software platforms. This type of software has paved the way for easy access to primary research.
Businesses can use online survey software and tools and to carry out all their survey research (save for creating the screener and questions). Many such tools available both allow you to build surveys along with deploying them.
To reiterate, market research surveys are powerful tools, in that they empower businesses to ask any question they choose to better understand their market and consumer base. They also can offer key insights into competitors.
The Components of a Market Research Survey
This tool contains two major components: the screener and the questionnaire. These form the bulk of the insights your primary research will gather.
There are also two auxiliary components to incorporate to make your survey research successful. These include the call-out (introduction) and the thank you message (conclusion).
Unlike the essential components, the need to use these will vary based on your survey deployment method and campaign. For example, an emailed survey won’t require a call-out, as the email itself serves this purpose.
A web or mobile survey, on the other hand, will need a call-out to get the attention of your respondents.
Here is a break-down of each component, beginning with the essential elements:
- The screener: Designed as a set of questions (like the questionnaire), this is the first stage of taking the survey. The screening questions are designed to determine whether a respondent is qualified to take the survey. You can set all the conditions for qualified participants.
- These conditions often deal with demographics, which is incredibly important, as you would need to first and foremost, survey your target market. The screener will ensure it is only your target market that takes part in the survey.
- The screener is often comprised of 2-3 questions.
- The questionnaire: The heart of the survey, the questionnaire is composed of a set of questions, which can be open-ended or close-ended. They can also exist in the form of ratings (starts or numbers).
- The questionnaire should ask all the necessary questions you need for a particular campaign or sub-campaign. Or, if used in a preliminary stage of your market research, they can deal with questions particularly designed to segment your target market.
- The call-out (introduction): A call-out introduces the survey to respondents in a number of ways. This element is the first the respondents will get in their survey experience.
- If respondents are contacted via email, the call-out is in the email’s body, inviting participants to take it, listing why it’s important, its length and what it’s used for.
- If the survey exists within a website (either as a banner, or button), the call-out is the clickable element itself (the button/banner to the survey). It too should explain the survey to respondents.
- If the survey is on a website/app, the call-out has to be visible and attractive enough for users to notice it and click on it.
- The thank you message (conclusion): When respondents complete the survey, this message should pop up to thank them for their participation.
- The survey often routes users to another page with a thank you message.
- It’s important, as it lets participants know that their survey has in fact been submitted.
How to Create a Market Research Survey
Given that there is much you can uncover with a market research survey, and many applications to use it for, it may be difficult to begin creating one. After all, you would need to tether it to the appropriate campaign to reap the most benefits out of it. You would also need to define its purpose.
Here are a few steps to take into consideration when starting on a market research survey project.
Step 1: Find a topic your business needs to learn more about.
This is particularly important if it is a topic that has little to no secondary sources. In this case, opting for a survey is the best way to learn more about it firsthand, from the people who matter most: your target market. Pay attention to any problems your business may experience, as surveys should help resolve them.
Step 2: Consider the topic in regards to your target market
When you’ve narrowed down a problem or two, think about your target market. Do you know who constitutes it? If yes, tailor your survey topic into a subtopic that they’ll be most likely to respond to. For example, if your target market is middle-aged men who watch sports, consider whether your problem/topic will be relevant to them.
If you don’t know your target market, you should conduct some secondary research about it first, then perform market segmentation (surveys can help on this front too).
Step 3: Find the larger application of the survey campaign
Now that you’ve settled on a topic/problem and decided on whether it’s fitting for your target market, consider what the parent campaign of the survey would be. Let’s hypothetically say your topic is related to a product. Would a survey on that topic benefit a branding campaign like finding your next slogan? Would it be better suited to settle on a theme for an advertising campaign?
Once you find the most appropriate application or macro campaign to house the survey, your market research will be organized and your survey will be better set up for success.
Step 4: Calculate your margin of error
A margin of error, in simple terms, is a measurement of how effective your survey will be. Expressed as a percentage, it measures the difference between survey results and the population value.
You need to measure this unit, as surveys represent a large group of people, but are made up of a much smaller group. Therefore, the larger the margin of error, the less accurate the opinions of the survey represent an entire population.
Step 5: Create your survey(s)
Now that you’ve calculated the margin of error, start creating your campaign. Decide on how many surveys you would need, in regard to your margin of error and your market research needs.
Start with a broader topic and get more specific in each question. Or, create multiple surveys focused on different but closely related subtopics to your main topic.
Send out your surveys through a trusted survey platform.
Questions to Ask for Various Campaigns
The steps laid out above are part of a simple procedure in developing a market research survey. However, there is much more to these steps, especially that of creating the survey.
Namely, you would need the correct set of questions, as they are the lifeblood of a survey. With so many different survey research campaigns and purposes, brainstorming questions can seem almost counterintuitive.
To avoid information overload and any confusion that creating a survey may incite, review the below question examples. They are organized per campaign type, so you can discern which questions are most suitable for which corresponding research purpose.
Questions for Branding
Branding campaigns include efforts that build the identity of your business; this includes gathering data-backed ideas on logos, imagery, messaging and core themes surrounding your brand. You can use these when embarking on a new campaign, revamping an existing one or when you’re looking to change your brand’s reputation and style.
- Which of these brands do you know?
- What do you like most/least about this brand?
- Which idea is more important? (Use an idea behind setting up your brand’s image/style)
- Which images do you find the most inspiring? (To compare images you’ll use in your marketing/ definitive to your brand)
- What do you like about [brand]? (Can be open-ended)
Questions for Advertising
Using market research for advertising will help you obtain ideas for new advertising campaigns, testing already established campaign ideas and predicting the success of new ones.
- How would you rate the motivating power of this ad?
- Which of the following ads resonate the most with you?
- Do you remember this ad? (Name and image/video of a popular ad within your industry)
- How do you feel after watching this ad?
- What kind of use do you think this product/service produces?
Questions for Comparing Yourself with Competitors
Studying your competitors is often associated with secondary research, but you can gain intelligence on this topic through your own survey research. The great thing about surveys is that you don’t have to focus on one competitor when managing these surveys.
- How often do you use this product/service?
- Which brand do you use for this product/service? (Include one open-ended answer).
- Which of the following products (same kind, different brand) do you find the most useful?
- What about [competitor product] would you like to see change?
- Which brand has improved your life? (Include one open-ended question).
Questions for Market Segmentation
This application is possibly the most challenging, as it involves understanding who your target market already is, then further segmenting it. We understand coming to terms with your target market first, before narrowing it any further down.
Here is how to segment your target market; you’ll notice that the questions are much more granular than the typical questions associated with each topic. (Ex: demographics typically ask for race, age, gender, income, etc).
- Demographic segmentation: Which of the following groups do you identify with most closely? (It can involve anything from music, to shopping habits, to lifestyle choices)
- Geographic segmentation: Which of the following areas do you typically spend time in to make physical purchases?
- Psychographic segmentation: How do you feel about retailers who test their products on animals?
- Behavioral segmentation: How often do you buy this kind of product?
- Sentimental segmentation: How do the following [practices, images, actions] make you feel?
Securing the Most Benefits Out of Your Market Research Survey
As we can deduce from this guide, the market research survey is a critical tool for market research. There is so much to discover about your industry, competitors and chiefly, your customers. But before making any hasty decisions, it is vital to peruse all your research documents, not just the primary research ones, such as surveys.
When you combine primary and secondary research sources, you’re setting up any business move for greater success.
That’s because market research involves studying more than one source. It may appear daunting, but with the right tools, you can design better products, innovate on existing products, appeal to a wider audience and gain more revenue from your marketing efforts.
Thus, pair your market research survey with other research means for a lucrative market research campaign. Knowledge truly is power.
Frequently asked questions
What is a market research survey?
A market research survey is a survey used for conducting primary market research and is the most commonly used market research method. Market research surveys help you understand your target market, gathering data necessary to make informed decisions on content creation, product development, and more.
What are the components of a market research survey?
There are 4 major components in a market research survey. First, we have the callout to get digital visitors to participate in a survey. Next is the screener which determines who is eligible to take the survey based on their demographics information and answers to screening questions. Then, there is the questionnaire—-- this is the heart of the survey, containing a set of open-ended or closed-ended questions. Lastly, there’s the callout. This introduces the survey to respondents. Next, there’s the thank you message. This acts as the conclusion to the survey.
How can you create a market research survey?
Creating a market research survey starts with identifying the topics your business needs to learn more about. Next, you consider topics within the context of your target market and find the larger application of the survey campaign. Calculate your margin of error and then create your survey using online software.
What types of questions should you ask on your market research survey?
You can ask branding related questions to gather information on how your identity of your business is perceived. You can also ask questions that spark ideas for new advertising campaigns. To supplement your secondary research on competitors, ask questions about your business’s place in the industry. Questions can also be used for market segmentation. These are questions on demographic, geographic, psychographic, behavioral and sentimental topics.
How can you get the most benefits out of your market research survey?
You can get the most out of your market research survey by using the correct online survey platform-- one with specific audience targeting for real consumers, radius targeting and quality screening questions-- you’ll get relevant answers from the right audience.
How to Build Effective Survey Studies for Valuable Market Research
How to Build Effective Survey Studies for Valuable Market Research
Survey research is an invaluable approach to primary research for any market research campaign. Effective survey studies allow you to observe virtually any topic as it relates to your target market, including a specific segment of your target market.
But with so many types of survey research campaigns and survey studies, it can be challenging to decide on how to pursue and form a survey study. After all, the goal with any data collection campaign is to extract data that is as accurate and reliable for your research needs.
This article expounds on how to build effective surveys from the ground up so you can proceed with your survey research with knowledge and ease of mind.
Finding the Macro-Application for Survey Studies
Every survey needs a purpose. Say you have a burning curiosity about your customer base. When tackling survey research, connect this curiosity to a more general-purpose for your business or institution (if you are a market researcher outside the business sphere).
Surveys can be applied to a vast number of macro-applications, i.e., the applications dealing with some category in the business or research sectors. First, find the most important topics (or said curiosities) that you would like to see your survey address. Then, categorize them in one of the following macro-applications:
- General Marketing: Marketing involves all the activities needed to promote a business. Marketing market research exists to help businesses gauge their campaign efficacy and better understand their customers. If you choose this application, consider how you can gain insights more deeply by choosing a subdiscipline within marketing.
- Advertising: Deploying sponsored messages to grow demand and elicit purchases, advertising is used to influence customer behavior. This involves prompting existing customers to make further purchases or acquire new customers. Surveys can be used to see which advertising messages are the most resonant and which ads spawn the most interest. Researchers can ask questions centered on comparing full ads, or parts of an ad such as the imagery, a video snippet or the copy.
- Branding: This discipline involves creating a reputation, an image and a set of associations around a brand. Branding helps brands differentiate themselves from one another, along with establishing a style that a company is easily remembered by. Businesses can tie their surveys to branding by creating surveys to test new logos, slogans, a unique value proposition, content ideas (for example, if a company that sells electronics seeks to attach lifestyle content to its branding) and more.
- Market Segmentation: This macro-application refers to studying customers closely by dividing a target market into smaller segments. After all, a target market includes all the customers most likely to buy from a particular business, but it is not solely defined by one group. At their core, business surveys are designed to understand customers to a T. With this said, researchers can form personal questions about their target market’s habits, lifestyles, preferences and more to distill them into several segments. From there, marketers can adopt different marketing campaigns for each segment.
- PR: Public Relations, or PR, as it is commonly referred to, aims to control the distribution and spread of information about a company (or individual) and the public. Its goal is to control the narrative of a business or organization to gain positive public perception. Surveys can help on this front in that researchers can design questions on how well respondents know a business and their general thoughts on its operations, products, experiences, performance, etc. Researchers can also test out press release ideas and pitches through these surveys.
Turning to Secondary Research
After filtering your curiosities and questions into a specific macro-application, you need to find all the available information surrounding this application as it relates to your survey subject. This means, before setting up your survey, researchers ought to turn to secondary research.
This is because you wouldn’t want to forego key data already conducted and made available. If you do, you’ll ask redundant questions, wasting both time, money and your survey on matters you could have found from secondary sources.
There are various secondary resources available online. These include webzines, trade publications, news sites and statistics websites. Additionally, research departments in universities launch their results online, which is especially if they cover your sector. You will need to visit these but the ones that study your particular industry and niche. MarketResearch.com is an efficient secondary source, as it covers timely reports across a gamut of industries.
Scrutinizing your competitors' digital properties is also a useful way to understand your target market, as it is shared (otherwise they wouldn’t be your competitors). B2B businesses in particular often publish reports on their industry, which often covers customer insights.
Researchers should collate and carefully organize the key and auxiliary findings they’ve gathered. To do this, creating a document to store all the information is necessary. The insights in the document will help in putting together surveys.
Preparing Preliminary Questions
After you’ve chosen a macro-application and gathered secondary information on it in relation to your industry and target market, you can advance to the preliminary question stage.
In this stage of building effective surveys, you should revisit some of the original curiosities and questions you wished to inquire of your target market. The most practical way to move forward is to cross-reference these original questions with the document of secondary research information.
Here is what to consider when doing so:
- Does any of the information you’ve found from conducting secondary research answer any of your original inquiries? If so, you won’t need to use the same questions in your surveys, unless you would like to extend the information from those questions. Perhaps the information you gathered answers only part of your question, or only about one segment of your target market.
- In these cases, it is apt to use the original question in your survey planning as a preliminary question. You can also evolve questions to make them veer in a slightly different, but not altogether different direction.
- Continue cross-referencing until you’ve gathered at least 10 questions you would like to see answered by your target market.
- Do any of them intertwine or focus on a similar topic? If so, consider grouping questions together, to determine if they’ll require being used in one or multiple surveys.
- When you’ve put together your preliminary questions, it’s time to contemplate the kind of study you’ll need to employ.
Choosing a Methodical Form of Survey Studies
Survey research entails much more than simply launching surveys through an online survey provider and waiting for results to pile in. Rather, it requires a more methodical approach, one with a particular timeframe and pipeline.
There are several forms of survey studies that are time-dependent. The following explains them:
- Retrospective Studies: Also called historical research, a retrospective study gathers data on occurrences that have already happened. As such, respondents discuss their past opinions, happenings and other memories in these surveys.
- Cross-Sectional Studies: A study in which research that gathers research about a targeted population at one fixed point in time. This type of survey research method is known as being a snapshot of a studied population.
- Longitudinal Studies: A study that gathers data on the same set of respondents over a period of time. This kind of research grants researchers the ability to closely examine the trajectories of their subjects over time (weeks to decades).
- Prospective Studies: A longitudinal cohort survey study that gathers data from similar respondents with a few dissimilar factors to determine how those factors affect a particular outcome.
Identifying the Correct Survey Research Method
After choosing the most suitable time-based survey study method, you need to identify another research method to carry out your survey studies. This kind of method deals primarily with the observational style and type of analysis you’ll need to conduct from your survey studies.
There are many different forms of survey research in this regard. The following lists the chief three such methods.
- Descriptive Research: This form of survey research is planned in advance and designed to extract data that can then be used for making statistical inferences on a target market. Aa such, it is considered conclusive and requires conducting quantitative survey studies.
- Exploratory Research: This kind of survey study is critical for the marketing and strategy aspects of a business. As opposed to gathering quantitative research, it focuses on discovering new ideas and insights, especially those pertaining to a target market and industry demands. In online surveys, exploratory research is often conducted via open-ended questions. This research method is qualitative, seeking to further grow a business and define company goals.
- Causal Research: Also quantitative and planned beforehand like descriptive research, this form is therefore deemed conclusive. Casual research seeks to discover the cause and effect between variables. It isn’t a form of observational research, as descriptive research is, however, as it sets to determine causal relationships via experimenting.
Opting for the Proper Survey Type for Your Survey Studies
When you have a steady strategy of the research methods your survey studies will apply, it’s time to adopt the proper survey type(s) for your survey study needs. Various survey types can be applied to different survey research methods.
In this regard, you can opt for survey types with your chosen research methods in a mix and match fashion. This is because different survey types are flexible; they have the potential to satisfy a bevy of research methods, including both time-based and analysis-based methods. The deciding factor for the survey types you implement is dependent on the subject of your study.
The following lists the different types of surveys to use in your studies. Several have their own sub-types of surveys.
- Customer Satisfaction Surveys:
- Gauge customer satisfaction with products, services, experiences & more.
- Includes the following subtypes: Net Promoter Score (NPS) surveys, Customer Satisfaction Score (CSAT) surveys, Customer Effort Score (CES), Visual RAtings surveys and custom surveys.
- Event Evaluation Surveys:
- Evaluate the experience and performance of participants in an event within the eyes of attendees.
- Can exist in print form (distributed at the event) or in online surveys.
- Brand Awareness Surveys:
- Measure how well your target market knows about your brand, along with providing opinions on its key features (logos, reputation, efficiency,etc.)
- Ideal for branding campaigns and before employing survey studies on advertising.
- Lead Generation Surveys:
- Purposed to gain contact information from your target market and reveal the types of individuals who make up your target market.
- Great for market segmentation and early research (before other survey types).
- Job Satisfaction Surveys:
- Used to understand how members of your target market feel about their jobs, a critical force in their identities and whether they can afford your product/service.
- Helps you learn which respondents are more satisfied with work/ income, thereby revealing which segment is more likely to spend.
- Employee Feedback Surveys:
- Used in businesses for internal purposes surrounding their own employees.
- Helps understand current pain points, successful and poor management styles, best HR incentives and how to improve processes and communication.
- Consumer Loyalty Surveys:
- Calculates how many and if members of your target market are loyal to your brand and your competitors.
- Helps brands reap customer loyalty.
Crafting the Questions for Survey Studies
Once you’ve chosen the most fitting survey types to use in your studies, you can finally move on to the survey itself. A sturdy online survey platform will allow you to get creative, granular and analytical with your survey production.
First, create a specific list of demographics and behavioral characteristics you will need to examine in your survey studies. Preset these requirements in the screening section of your survey tool. You should set quotas to assure you’re obtaining the appropriate respondents in your survey.
Next, consolidate your preliminary questions with the new ones you’ve come up with while identifying the correct research methods. These oftentimes influence the kinds of questions you’ll need to ask. This also requires organization, as certain questions will belong on different surveys.
If you’re struggling to form relevant and useful questions, read our guide on writing survey questions. When you’ve come up with questions, considering adding layers to your survey. For example, a particular answer that one respondent answers with may require a different follow-up question than that which another respondent answers with.
Sometimes, this requires creating different question paths for different types of answers. You can achieve this by applying advanced skip logic into your survey. Your online survey tool of choice should allow you to add media files (images, GIFs, video snippets) to make your survey more engaging.
Remember to keep your surveys so as to avoid survey attrition. Now that you’ve come up with the questionnaire questions, review your entire survey. Make sure you’re asking the questions that will help flesh out your survey studies.
Once you do that, you’re ready to launch your survey.
Other Considerations for Survey Studies
There are several things you need to consider for your survey studies, most of which are dependent on the online survey platform you use. These involve ease of use, respondent capacity, publisher networks (where the surveys will be deployed) and many more user experience (UX) capabilities.
For example, in the aforementioned survey types section, there are dozens of surveys you can form for both research and business purposes. It is key to use a platform that can provide structures and elements for all, or at least for the kinds you need.
As for ease of use, assess the difficulty in using an online survey tool. A strong survey platform allows you to make a survey in three easy steps. When you thoroughly vet a survey platform, you can objectively decide which is best for your survey studies.
Frequently asked questions
What is survey research?
Survey research is the process of performing research through the use of surveys. After the survey data is collected from respondents, it is analyzed in order to draw conclusions about the topic at hand.
What are some of the macro applications of surveys?
Surveys can be used to understand many broad aspects of business or research including general marketing, advertising, branding, market segmentation, and public relations.
What is secondary research?
Secondary research is the process of gathering information that has already been conducted and is made available for collecting and studying.
What is descriptive survey research?
Descriptive research is conducted to explain the characteristics of a sampling pool or a study of an issue. It is a form of survey research that is performed by conducting quantitative surveys in order to extract data that can be used to make statistical inferences.
What is exploratory survey research?
Exploratory research is the earliest form of research conducted around a subject. It is used in order to identify new ideas and insights, particularly about a problem not clearly defined for further studies, such as descriptive research. It is done by conducting qualitative studies in order to gain new information about the subject.
The Complete Guide to Qualitative Market Research
The Complete Guide to Qualitative Market Research
Qualitative research is one of the most prominent research methods in the ever-increasing research sphere. Running counter to quantitative research, qualitative research encompasses a distinct set of differentiating qualities (no pun intended). These attributes prove that these two methods ought not to be used interchangeably.
So what exactly is qualitative research? At a glance, this type of research method seeks to gather in-depth data about a phenomenon without focusing on numerical data or on quantities.
But there is much more to this kind of study method. Learn holistically about qualitative market research with this complete guide.
What Defines & Makes Up Qualitative Research?
Qualitative research is centered around experiences, ideas and opinions. As such, it does not focus on statistical or quantitative outcomes. Instead, it seeks out an in-depth understanding of an issue, occurrence or phenomenon.
Thus, this research method zeroes in on the “what” and more importantly, the “why” of a research subject. (Unlike quantitative research, which focuses on the “how much”).
Here are some of the applications of qualitative research:
Understanding an issue in greater depth
Finding the reason behind an occurrence (whether it’s desirable or undesirable)
Uncovering trends in target market opinions
Forming educated solutions to address customer/studied subject concerns
Discovering the causes of certain actions
Qualitative research generally relies on a smaller sample size in order to get a deep read of happenings, causes and motivations. This kind of research method functions through the usage of open-ended and exploratory questions.
Understanding the “why” behind an issue is then used to make decisions on how to resolve the issue or how to improve on an existing productive situation.
Qualitative data must occur in natural environments. This denotes a kind of environment in which participants discuss their opinions at length and at ease, which researchers use to gain deeper knowledge and form inferences around a topic.
Prior to the internet, this kind of research was conducted in-person, but with the advent of the internet and innovations in market research, qualitative data has been collected online. The digital space can also serve as a natural environment.
The Five Main Types of Qualitative Research
Just as with quantitative research, there is not a single approach to conducting qualitative research. On the contrary, there are five main varieties of performing qualitative research. Aside from their methodology, these sub-categories also seek different types of answers and conclusions.
1. Narrative Research
This research is used to form a cohesive story, or narrative, by way of consolidating several events from a small group of people. It involves running in-depth interviews and reading up on documents featuring similar actions as a means of theme-searching.
The point of this is to discover how one narrative is shaped by larger contextual influences. Interviews should be conducted for weeks to months and sometimes even for years. The narrative that the researcher uncovers does not have to be presented in sequential order.
Instead, it should be projected as one with defined themes that attempt to reconcile inconsistent stories. This method can highlight the research study’s ongoing challenges and hardships, which can be used to make any improvements.
2. Ethnographic Research
The most common qualitative research method, ethnography relies on entrenching oneself in various participant environments to extract challenges, goals, themes and cultures.
As the name suggests, it involves taking an ethnographic approach to research, meaning that researchers would experience an environment themselves to draw research. Using this firsthand observation, the researcher would not need to then rely on interviews or surveys.
This approach may seem to be far-fetched where market research is concerned, but it is doable. For example, you’d like to see the effectiveness or frustration that customers face when using your product. Since you can’t follow them home, you can request videos that show them using it. Many big brands have call-outs on their websites (ex: on product pages) for their customers to send in videos of their interactions with the products.
3. Phenomenological Research
This qualitative method entails researchers having to probe a phenomenon or event by bringing lived experiences to light and then interpreting them. In order to achieve this, researchers use several methods in combination.
These include conducting surveys, interviews and utilizing secondary research such as available documents and videos on the studied phenomenon. Additionally, as in ethnographic research, phenomenological research involves visiting places to collect research.
These will help you understand how your participants view your subject of examination. In turn, you will gain insight into the participants’ motivations.
In this research type, you would conduct between 5 and 25 surveys or interviews, then peruse them for themes. Once again, you would scrutinize experiences and sentiment over numerical data.
4. Grounded Theory Research
In contrast to phenomenological research, which seeks to fully form the core of an issue, grounded theory attempts to find explanations (the why) behind an issue. To achieve this, researchers use interviews, surveys and secondary research to form a theory around the issue/occurrence.
The sample of this study tends to be on the larger side, at 20-60 participants. Data extracted from this type of research is interpreted to determine the reasoning behind, for example, heavy usage of or frustration with a product. These types of studies help a business innovate an existing product by getting into the weeds of how it’s used.
5. Action Research
This type of research involves researchers and participants working collaboratively to bring theory to practice. Also called participatory research, collaborative inquiry, emancipatory research and action learning, this method entails the act of “learning by doing.”
This means a group of researchers come together to find and address a problem, resolve it and then study the success of their endeavors. If they underperformed or their outcomes don’t satisfy their expectations, they would then reattempt the process.
In action research, a researcher spends a considerable amount of time on collecting, analyzing, and presenting data in an ongoing, periodic process. This involves researchers coming up with their own surveys and interviews around a subject matter, then presenting their findings to one another to draw conclusions and solutions.
They would put into practice the means to improve a situation and continue measuring their success throughout the process.
Examples of Questions for Qualitative Research
When working within the capacity of any of the above research types, it’s crucial to ask the right questions. Here you’ll find the questions you can use when conducting each of the five types of qualitative research.
Bear in mind that some of these questions will appear to be similar in nature; some are even interchangeable. That is normal, as researchers may search for the same answers, but apply a different approach in their research method.
In any case, all of the below features questions that fit within the larger qualitative research framework.
Learn more about asking insightful market research questions. Here are a few examples of the questions within the five categories:
1. How do people who witnessed domestic violence understand its effects in their own relationships?
Variable: Views of domestic violence on one’s own relationships
Demographic: People in relationships, who’ve witnessed domestic violence
Qualitative Research Type: Narrative
2. What are the lived experiences of working-class Americans between the ages of 20 and 40?
Variable: Experiences and views of a working-class background
Demographic: Working-class Americans ages 20-40
Qualitative Research Type: Narrative
3. How do Asian Americans experience reaching out to address mental health concerns?
Variable: The experiences in seeking out care for mental health
Demographic: Asian Americans seeking help for mental health
Qualitative Research Type: Ethnographic
4. What do you enjoy about this product or service?
Variable: The positive experiences of using a particular product/service
Demographic: The target market of a product or service
Qualitative Research Type: Ethnographic
5. How have people who have experienced poverty changed their shopping habits when they entered the middle (or higher) class?
Variable: The changes or stagnation in shopping habits
Demographic: those who experienced poverty, but climbed the social ladder
Qualitative Research Type: Phenomenological
6. What was it like when you had a negative online shopping experience?
Variable: unpleasant shopping experiences
Demographic: a group that is most likely to shop at a particular online store
Qualitative Research Type: Phenomenological
7. What influences managers in private sectors to seek further professional advancement?
Variable: Motivation for seniority
Demographic: Managers in the private sector
Qualitative Research Type: Grounded Theory
8. How do women in third world countries set up financial independence?
Variable: Efforts at reaching financial independence
Demographic: Women in third-world countries
Qualitative Research Type: Grounded Theory
9. What impact does collaborative working have on the UX optimization efforts of a telecommunications company?
Variable: effects of collaboration on the UX of a telecommunications company
Demographic: workers in the telecommunications space
Qualitative Research Type: Action Research
10. What strategies can marketing managers use to improve the reach of millennial customers?
Variable: Strategies to improve millennial reach and their outcomes
Demographic: Marketing managers
Qualitative Research Type: Action Research
When to Use the Research and How to Analyze It
The qualitative research method has specific use cases. You ought to consider which is best for your particular business, which includes your strategy, your marketing and other facets.
The core of qualitative research is to understand a phenomenon (a problem, an inadequacy, and a slew of other occurrences) including its causes, its motivations, its goals and its solutions. Researchers do this by observing smaller portions of a population.
Researchers should use this form of research whenever you need to get the gist of a particular occurrence or event. It is particularly useful for studying how your target market experiences certain situations and how it feels about them.
There are several more specific ways that elucidate why this research style is valuable if not completely necessary. Here are some of the most crucial ways this method of research is vital:
Helps brands see the emotional connections customers have with them
Allows brands to find gaps in customer experience (CX) and user experience (UX)
Enables brands to create experiences that are more tailored to their target market
Helps businesses understand how they can improve on their product, service or CX
Finds experiences that customers had that highlight sensitive topics/language for them
Shows businesses how customers compare them to their competitors
Identifies possible solutions and innovations based on customer attitudes and experiences
To analyze qualitative research, you should first identify your subject of study and decide on the type of research you need to conduct based on the five types of research that fall under the qualitative category.
Then, brainstorm several questions that you can use to form the base of your studies. During the process make sure to jot down (either digitally or otherwise) your observations. For example, record interviews and store surveys in an organized database.
Make sure you ask open-ended questions in surveys, interviews, focus groups, et al. Aggregate secondary research such as government database documents, articles in your niche, images, videos and more.
Search for patterns or similarities within your findings. When you group them together and organize them by demographics, you can start drawing conclusions and proposing solutions.
The Benefits and Drawbacks of Qualitative Research
Qualitative research can be extraordinarily beneficial. But as with other aspects of research and beyond, it too comes with a set of drawbacks. As a business owner, marketer or market researcher, you should know both the pros and cons. Here are some notable ones:
Benefits
More intimate understanding of context and causation: besides understanding “what” in a granular way, you also learn the “why” and “how” of a particular situation.
Understanding key experiences: Open-ended questions lead to unique answers, exposing things numerical-based surveys can’t answer.
A foundation of deep insights: The design of the study is made to understand how customers relate to particular occurrences, events, ideas and products.
Context-driven: Finding insights on motivation and past behaviors allows researchers to understand what their target market needs and what it tries to avoid.
No need to find and create the correct measuring units: Open-ended questions don’t require a scale, a number range or any other measuring tools — one less thing to worry about.
Smaller sample size: Smaller sample sizes allow researchers to study responses more thoroughly to form more accurate hypotheses and conclusions.
Inspirational: The responses received can also help researchers form new studies.
Flexible and detail-oriented: Since questions aren’t based on scales and other units, you can ask more creative and in-depth questions. Questions focus on details and subtleties for robust insights.
Drawbacks
Relies on researcher experience: It relies on the researchers’ experience; not all are familiar with industry topics.
Not statistically representative: Only collects perspective-based research; does not provide statistical representation. Only comparisons, not measurements can be executed.
Difficult to make copies of data. Individual perspectives make it hard to replicate findings, making it it more difficult to form conclusions.
More likely to have researcher bias: Both conscious or subconscious of the researcher can affect the data. The conclusions they draw can thus be influenced by their bias. (This can be avoided by using controls in data collection.
The Final Word
Market research is a wide-spanning undertaking. It has a wide swath of aspects, practices and applications. As such, researchers should know its main categories and qualitative research is one such category of significance.
As opposed to quantitative research, which has four methods, qualitative research has five — not all of which will be of use to your particular market research needs. In any case, this type of research involves imbuing as much context and particularities around a phenomenon as possible.
As such, researchers should create questions more specific to the aforementioned examples of this article. That is because those are more encompassing, generalized questions that researchers can attempt to answer after conducting all of their research and parsing of the findings.
But prior to that, researchers should ask several related questions around a particular topic and tailor those questions as best as possible to the target audience.
Frequently asked questions
What is qualitative research?
Qualitative research is a type of research that is conducted to gain deep or unexpected insights rather than focusing on numeral or quantitative data.
Why is qualitative research conducted?
Qualitative research is conducted to find the “why” of the research subject, rather than the “what’ of that subject. For example, qualitative research might be conducted to understand an issue more deeply, to understand why something is happening, or to learn how to address a target market’s concerns.
What is narrative research?
Narrative research is a type of research that is used to create an in-depth story about a phenomenon or event. It is conducted by interviewing a small group of people who were directly involved in the event.
How is ethnographic research conducted?
When conducting ethnographic research, the researchers use firsthand observations of an environment to more deeply understand the goals, challenges, or opinions of the target audience.
What is action research?
Action research is a type of qualitative research in which researchers and participants collaborate to better understand a phenomenon. Together the group works to find and solve the problem by gathering information on an ongoing and evolving basis.
The Complete Guide to Quantitative Market Research
The Complete Guide to Quantitative Market Research
Quantitative research is a chief category in the research sphere, along with qualitative research. An encompassing aspect of market research, it can include both primary and secondary methods of extracting data.
Although used interchangeably with qualitative research, quantitative research is a distinct process that should not be confused with its counterpart. In fact, it is the opposite of qualitative research.
Let’s navigate through the waters of quantitative research in this complete guide.
What Defines & Makes Up Quantitative Research?
As its name suggests, quantitative research is the process of aggregating quantitative, or numerical data for research purposes. This data is used for a number of applications. These include:
- Quantifying opinions, behaviors, attitudes and problems
- Making generalizations
- Forming predictions
- Discovering patterns
- Determining averages
- Testing relationships
Quantitative research generally relies on a larger sample size in order to quantify any issue or variable. In order to achieve this, this research method involves using mathematical and statistical means.
This type of research answers the “what” and the “how much” of a subject within a research endeavor. As it forms generalizations, this type of method involves surveying a larger population, using measurable data and processing all the data first and then analyzing it from a statistical standpoint.
The Four Main Types of Quantitative Research
There are four main ways to perform quantitative research. Aside from their methodology, these sub-categories also seek different types of answers and conclusions.
1. Descriptive Research
This is used to determine the state of variables. It describes the situation and environment surrounding a variable or topic. As such, it is used for arranging comparisons, outlining sample characteristics, overlooking emerging trends and confirming existing phenomena.
The data is collected by way of observation. Descriptive Research is used to form a hypothesis, but only after having aggregated all the necessary data.
2. Correlational Research
This research method is used to examine the relationships between different subjects and variables. Analyzing relationships is necessary to either test a hypothesis or a prediction. Because this research focuses on relationships between fixed variables, other outlying variables are not part of the investigation.
Correlational research is in direct opposition to experimental research, as none of the studied variables are manipulated. Correlations can be either positive or negative, with different degrees of the relationship’s strength.
3. Experimental Research
This method is used for finding whether there is a cause and effect relationship among variables. This kind of research relies on the scientific method. Unlike correlational research, experimental research involves manipulating variables.
Researchers would manipulate a variable to uncover its effect on another one. This method is frequently referred to as true experimentation, as no experimental undertaking leaves all variables unchanged; at least one must be influenced in some way.
This includes manipulating, randomizing or reverting back a variable. The variables are then measured, calculated and compared.
4. Survey Research
The final research method is crucial to understanding behavior. In market research, it is often used to acclimate a brand with its target market’s desires, needs, points of contention and behaviors.
Surveys allow researchers to ask pointed questions to either discover their target audience or get a granular sense of their opinions. As such, they can be conducted within one group or many, for the sake of comparison.
Instead of turning to survey panels, which are likely to have skewed or biased results, researchers should use a random sample of people. A non-panel-based survey will garner more respondents that aren’t motivated by professional compensation.
Surveys can be administered by mail, in-person, on the phone, or digitally using various market research tools. Digital methods offer even more options, including online surveys, third-party platforms, email, and in-app surveys.
Examples of Questions for Quantitative Research
Survey research has a far larger scope of questions than do the other three types, as researchers can ask practically anything to conduct their studies. However, there are some best practices in survey questionnaires, such as focusing on your industry, your product and the desires of customers.
Learn more about asking insightful market research questions. Here are a few examples of quantitative research questions in the three other categories.
- Is working from home the best option to improve productivity for employees with long commutes?Variable: Working from home and in-office
Demographic: Employees with long commutes
Quantitative Research Type: Experimental - How has the coronavirus changed employment for white-collar workers?
Variable: Employment types and statuses
Demographic: White-collar workers
Quantitative Research Type: Experimental - How often do working people travel for a holiday?
Variable: Amount of times respondents travel during a holiday
Demographic: working people
Quantitative Research Type: Descriptive - How much would you pay for a subscription to an entertainment magazine?
Variable: payments for a magazine subscription
Demographic: women aged 14-44, those interested in celebrities
Quantitative Research Type: Descriptive - What is the difference in smartphone usage between Millennials and senior citizens?
Variable: Time spent on using a smartphone
Demographic: Millennials and seniors
Quantitative Research Type: Correlational - Does the leadership style of car shop owners predict the job satisfaction of car salespeople?
Variable: Leadership style and job satisfaction
Demographic: Car shop employers and salespeople
Quantitative Research Type: Correlational
When to Use Quantitative Research and How to Analyze It
The quantitative research method has specific use cases. You ought to consider which is best for your particular business, which includes your strategy, your marketing and other facets.
The core of quantitative research is to quantify a phenomenon (a problem, an inadequacy, and a slew of other occurrences) and understand its prevalence. Researchers do this by observing large portions of a population.
You should use this form of research whenever you need to be presented with the state of things at a higher level, or from a bird’s eye view. This Is because this type of research can identify links between various factors, look for correlations and discover cause and effect relationships.
Researchers can then use the results of their findings to form predictions. This is useful in market research when launching a new product, brainstorming product ideas or innovations or growing a customer base.
To analyze this research, it should first be made quantifiable and objective. Researchers should pin down the scales and units of measurements in their various studies. Then, they should organize them into easily interpretable formats.
For example, once you gather the numerical data, you can enter it into a spreadsheet. Thereafter, you can organize it by desegregating it into graphs, charts and tables. Finally, you should draw data-based conclusions from your study. You can also do further sleuthing via advanced analytics, which is made easier using a survey software for market research.
The Benefits and Drawbacks of Quantitative Research
Quantitative research has a bevy of benefits; it also has some hindrances. You should peruse both the positive and negative qualities of this research type before setting out on any major research project. The following may help you choose one form of research over the other, or use aspects of both.
Benefits
- Larger sample pools: the larger the group of respondents, the more accurate are the results.
- Highly structured: Surveys, questionnaires, and other tools for recording numerical data
- Focused: The design of the study is determined before it begins
- Theory-based: Research tests a theory to provide support/proof
- Designed to Be Analyzed: Numbers/statistics exist as tables, charts, figures and other non-textual forms for easy analysis.
- Objective: Steering clear of bias as the research is separated from the data & only objective responses are sought.
- Direct comparisons of results: The study can be set in different cultural environments, times or different groups of participants with a statistical comparison of results.
Drawbacks
- Focuses solely on numbers: This can be limiting as researchers may overlook other data and larger themes.
- Superficial Representations: It cannot adequately describe complex concepts (ex: feelings, opinions) it only shows the numbers behind them.
- Several factors can invalidate results: A hypothesis and a model for collecting/ analyzing data.is required; any mistake can lead to bias and inaccurate illustrations.
- Erred Structure: If any data is missing or if measurements are not clear, biases easily take precedence.
The Final Word on Quantitative Research
Market research is far too encompassing to fully complete, especially in a limited amount of time. To tackle market research, begin with a research method. Quantitative research is often a good starting point to get survey responses, as it shows you the existence of a problem by way of quantifying it.
Aside from confirming the existence, it can help confirm a hypothesis, find correlations and prove cause and effect relationships. A hard set of data can also help you make educated predictions.
While the three types of quantitative research methods are useful, they do have several disadvantages. The fourth one, ie, survey research helps fill in the gaps and inadequacies of numerical limitations. Interestingly enough, they too can be a source of hard data and numbers.
Either way, market research is sure to benefit from incorporating surveys as part of the processes.
Frequently asked questions
What is quantitative market research?
Quantitative market research utilizes the techniques of quantitative research in order to better understand the target market. In quantitative research, the information gathered from surveys and questionnaires is converted into numerical values so it can be easily analyzed.
What types of questions do quantitative research answer?
Quantitative research seeks to define “what” and “how much.” It is used for identifying patterns, making predictions, establishing averages, and quantifying opinions, attitudes or behaviors.
What are the four main types of quantitative research?
The four main types of quantitative research are survey research, correlational research, descriptive research, and experimental research.
What type of surveys are used for quantitative research?
Quantitative surveys are best suited for quantitative research. In this type of survey, there are no open-ended questions, and all responses can be assigned a numerical value. In most cases, a quantitative survey is distributed to a large and random sample of individuals.
Why are large sample sizes important when conducting quantitative research?
A small sample size can lead to inaccurate results. The larger the sample size (i.e. the group of individuals who receive the survey), the more likely it is that the results will be statistically significant and accurate.
Your Ultimate Guide To Writing Amazing Survey & Poll Questions
Contents
Your Ultimate Guide To Writing Amazing Survey & Poll Questions
Whether it is your first time creating a survey on your own, or you are a seasoned research professional, one thing is for sure: if you can’t write good survey questions, you won’t get helpful consumer insights.
But just what is a “good” survey question anyway? In this guide, we break down the four key elements that you need to master in order to write better survey questions.
A “good” survey question is:
- One that achieves your survey and data goals.
- One that is clear and direct.
- One that carefully considers user experience.
- One that is free from bias.
Throughout this guide, we will show you the tactics you can use to make sure you are delivering quality survey questions. To help you along, we will also show you what not to do, so you can easily spot the difference between a good question and one that needs some work.
For example, let’s say we want to avoid bias. Which of these is the better choice?
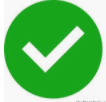
This is an example of a direct, straightforward question that has eliminated bias. Nice work!
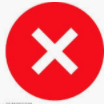
This is an example of Prestige Bias. By letting the respondent know that an authoritative source says smoking causes cancer, you are biasing the respondent to agree with the source.
Try out these question quizzes at each section to test your knowledge as you go. Let’s get started.
Set (and Achieve) Your Survey Goals
Everyone wants to write good survey questions–they want to be clear, direct, engaging and get respondents excited about answering.
But these are not the most important reasons to take care when writing survey questions.
The reason is if you build a survey with the wrong questions or question types, you could get stuck with a bunch of data you can’t use.
That’s why it is important to set data goals in advance and tailor your survey to achieve those goals from the very beginning.
How To Set Survey Data Goals
On the surface, this may seem simple: just state what you want to learn from your respondents and go from there.
But different surveys deliver different types of data. So you need to know how you plan to use the data you get from your questions first.
For example, let’s say your goal with your survey data is to create a map so you can continuously survey consumers every month and map their responses over time.
For this to work, you will need to combine demographic data with raw numbers of respondents and their answers.
You also want to ensure that your questions are simple and not prone to shifts in understanding based on geographic location or time passing.
Knowing these data goals, which of these two questions would be better for your survey?
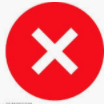
By adding a specific type of summer location, you assume that your respondents go to the beach or have been recently enough to have a favorite activity there. This will bias your survey towards people that live closer to a beach. Also, by allowing an open-ended question at the end, you will make one answer choice unusable for your map.
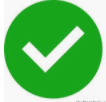
By keeping responses general, you avoid bias towards one geographic area. And by having a fully quantitative survey but also including a “Something Else” answer choice, you don’t force your respondents to give an answer that doesn’t apply to them, while still ensuring you only get data you can use for your map.
In order to discover the question types that may work best for your survey goals, check out this sample results page. Do an export of the data so you get a sense of what you will have to work with once your survey is completed.
Next, you’ll want to check with your stakeholders to ensure that you are aligned on the questions you want to get answered, how you want to use and ingest survey data and what you are hoping to be able to deliver at the end.
How To Ensure You Meet Your Goals
With Pollfish, you have 11 different question types to choose from. All of these question types deliver data slightly differently.
It’s also critical to deliver these questions in a structural order that makes sense to the respondent and reduces bias from their answers (more on that later).
Depending on your survey goals, you’ll want to choose these questions wisely to ensure you have a data set you can use.
The most important difference between survey question types is the difference between Quantitative and Qualitative research.
From Qualitative vs Quantitative survey questions
Quantitative research is about collecting information that can be expressed numerically. Researchers often use it to correlate data about specific demographics, such as Gen Z being more likely to focus on their finances than Millennials. Quantitative research is usually conducted through surveys or web analytics, often including large volumes of people to ensure trends are statistically representative.
Even when the survey audience is very large, quantitative research can be targeted towards a specific audience, usually determined by demographic information such as age, gender, geographic location.
Qualitative research focuses on personalized behavior, such as habits or motivations behind their decisions. This can be gathered through contextual inquiries or interviews to learn more about feelings, attitudes, and habits that are harder to quantify but offer important additional context to support statistical data.
When quantitative and qualitative research are paired, a complete set of data can be gathered about the target audience’s demographics, experience, attitudes, behaviors, wants and needs.
Based on this description, can you tell which is an example of a qualitative question type?
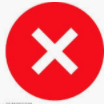
Qualitative surveys don’t have set answer choices. Instead, they allow respondents to speak in their own words about experiences with your product or service.
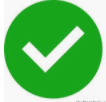
Qualitative survey questions allow respondents to speak in their own words about your product or service without selecting from pre-selected answer choices. You can use these questions to gain a deeper understanding about your consumers and their feelings towards your product outside of what you’d normally ask them. Feel free to ask more broad questions and let them open up.
But differences between quantitative and qualitative are not the only differences between question types. You can still gather a broader range of emotions from respondents without going to a fully qualitative survey.
Question types like matrix questions, where respondents can rank the importance of different product or service features can provide more quantifiable data while still giving a broader range of interest than a simple one-by-one ranking.
Lastly, some researchers choose to combine qualitative and quantitative research. By allowing an open-ended “other” field to single selection questions, for example, researchers collect quantifiable data, while still allowing the option for further context.
Based on your research goals, this added field can either provide needed context for deeper understanding of your audience, or create unnecessary noise that can’t be quantified and throw off the data set.
It all depends on you. So think it all the way through and have a plan in place before you begin creating your questionnaire.
Question Writing 101: Clear, Direct, Well-Tested
When writing anything, clear, direct communication should be the ultimate goal. You want to ensure that, regardless of any flowery language or interactive content features, the core of what you are trying to say is communicated loud and clear.
With survey questions, this becomes even more important.
Once you have selected your survey goals and you have a good idea of what kind of data you want to collect, the goal of your survey questionnaire is to get respondents to completely fill out your survey as accurately as possible.
Market research best practices show that it is best to let respondents decide if they want to finish your survey or not. With Pollfish, respondents can escape the survey at any time. Therefore, all responses are voluntary and of higher quality than if respondents were forced to finish the survey.
What that means for you as the researcher is you have to ensure that your questions don’t drive respondents away.
Don’t worry, Pollfish is here to help you with that. First of all, each questionnaire is reviewed by our research experts prior to launch. But you can help by following these three steps to survey question writing: be clear, be direct, and test on real people.
Be Clear
In order to get the types of answers you expect, you must ensure that your target audience will fully understand what you want from them.
What does that mean? Below are a few common mistakes researchers make when writing their first questionnaire.
Too Much Jargon
When writing for a niche audience, industry jargon can be a welcome addition. Things like common abbreviations and loaded terms help build rapport and allow you to establish a personal connection with your readers.
Not so in market research.
Imagine you are reading an article and you come to some jargon you don’t recognize. All it takes is a quick Google search and you have learned some new terminology.
But if you come to a survey question that contains an acronym or abbreviation you don’t recognize, not only will you feel like you can’t answer that question, you will feel like maybe you aren’t qualified to answer the rest of the survey. You may attempt to guess at the meaning, or escape the survey all together.
Even if your audience is screened to only be in your industry, don’t assume everyone uses the same terminology you do. Take care when using any kind of jargon, acronym or abbreviation in your survey questions.
Extra Explanation
Most survey question types are fairly self explanatory. If there are 5 answer choices and the instruction is “Select One”, there isn’t much room for interpretation there.
But when questions get more complicated, extra explanation is essential.
For complicated concepts or ideas that respondents may only have some ideas about, a “description” question type can be helpful in clarifying which part of the idea a respondent should focus on.
Matrix questions are one question type where you have to be careful of your labeling and explanation. Let’s take a look at two Matrix questions and see which is the most clear.
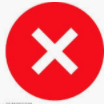
How are your respondents supposed to know if 1 is good or bad? When you use matrix questions, ratings scales and any other rating or ranking question, be sure to label questions clearly.
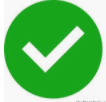
As you can see, everything here is labeled so it is very clear for each amenity what you are choosing when you select each answer. When you use matrix questions, labels are very important. From the row titles to the answer choices, make sure it is very clear what respondents are selecting.
Questions that contain media like images, audio files and videos also often require further explanation. Let’s take a look at the video questions below and see which is the most clear.
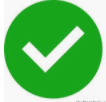
With questions containing required media, it is always a good idea to give explicit instructions as to what to do with that media. Once you have done that, you want to be very specific with what you ask respondents to deliver. If your ask is too vague, you are likely to get a lot of short, unthoughtful responses.
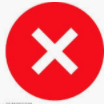
When you use Pollfish for video questions, respondents are required to watch the entire video before answering. Still, you want to be clear about what you want. If you want them to watch the entire video, say so. Also, be specific with what you want them to pay attention to. Otherwise, you are likely to get a lot of vague, unthoughtful responses.
If it is necessary to consume all media to understand the question being asked, make sure your instructions clearly state this. You can even include your media as a separate question.
Create non-leading questions
It can be tempting to write questions in a way that seems obvious to you, but survey questions should not follow the same patterns we have when speaking to each other.
Although plain language is advised, inserting your own opinions—even subtly— into the survey question can lead respondents to feeling more inclined to agree with the answer you want.
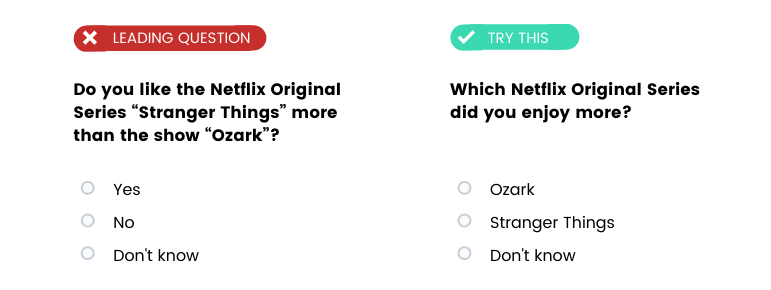
The second question remains neutral towards either show. Since the goal of your research is to uncover the most accurate data, you want to avoid pushing respondents towards one answer over another.
Make Answer Choices Clear & Distinct
Once you have made sure your questions and instructions are clear and direct, make sure your answer choices are distinct from one another.
One common reason respondents bounce from a survey is they cannot decide between two similar-seeming answer choices.
This can also reduce data quality because it may result in respondents offering answers they don’t really mean.
Although “yes” and “no” can seem like two distinct answer choices, these also don’t offer gradients within the answer options to appeal to respondents who don’t feel strongly or are unsure of an answer.
We recommend avoiding “yes/no” questions in favor of a Likert Scale or by writing answers out entirely to ensure less ambiguity and reduce bias.
Which of these questions seems correct?
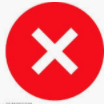
This question not only assumes the respondent drinks beer, but drinks beer enough to know the difference between these varieties. As a researcher, you don’t even know at this point if your respondent drinks at all. Answer choices need to be clearly distinct to all potential respondents, unless you have narrowly filtered your audience to ensure their knowledge.
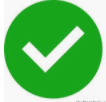
While this question is not as specific as it’s counterpart, it allows all possible respondents a chance to answer, while even making concessions for those who don’t drink at all. Once respondents answer here, you can get more specific (while ensuring the ability of respondents to participate) by using skip logic. Have the respondent answer and, for the ones who select beer, send them on to a question about which variety they prefer. That way, you can be more assured they will know the difference.
Be Direct
Good survey questions contain two parts: the question and the instructions.
For many Pollfish survey question types, some level of instruction is built in. But you want to be sure that when you deliver instructions, you are speaking directly, in clear, declarative statements.
Using too much passive language and indirect phrasing can create unnecessary confusion. Let’s take a look at the two examples below. Select which you think is the most direct.
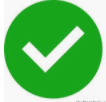
While these two questions may seem to say the same thing, using softer, more imprecise language opens up the potential for confusion. Using very direct, plain-spoken language removes any such ambiguity. Or to put it more simply, it makes questions (nearly) idiot-proof.
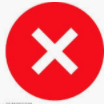
While these two questions may seem identical, the indirect language here leaves room for confusion. For example, who am I choosing for? Without a direct subject, I am left to wonder why I might be inclined to choose one flavor over another. Maybe when I buy ice cream, I only buy it for my husband because I am on a diet. If you want your respondent’s personal preference, ask for it, as clearly and directly as possible.
Always keep your Survey Goals in mind
As we learned in part 1, survey goals are at the core of all research projects. To make sure you’re writing the right survey questions to meet the right goals, consider the flow and structure of the survey and how the respondent will be presented with each question. Your goals may also dictate the type of question respondents should be given.
Example: Survey goal is brand awareness
It’s best to begin a brand awareness survey with an open-ended question for respondents to recall brands that are top-of-mind, prior to being presented with a series of questions that might trigger their recall.
If the survey goal is press for a specific cereal brand, ranking questions can help uncover consumer preferences.
Ranking questions ask respondents to order answer choices, forcing them to choose one over another. These are best for establishing stronger opinions or validating a strong preference.
The data from a ranking question could be “85% of consumers believe that Trix is the best children’s cereal”, providing a good headline for a research story.
Matrix questions allow respondents to apply a ranking scale towards each cereal, but don’t force them to choose one over another or compare them. These are a better question choice to measure other sentiments.
The possible data to come from a matrix question could be that nearly ¾ of 90’s kids recall Frosted Flakes’ “Tony the Tiger”, however, the Cheerios bee has largely been forgotten.
Test On Real People
While it is important to align with all stakeholders internally on survey goals, you also want to test out your final questionnaire on some real people.
This can mean either sharing it with co-workers who are not familiar with the research project, or emailing your questions to a friend.
Getting outside help can be essential in identifying unclear directions that cause confusion for your respondents.
Respondent Experience: Think Like An App User
Because nearly everyone has an internet-enabled device with them at all times, survey companies have identified the opportunity to use this technology to reach respondents.
Unfortunately, not all surveys are created equal when it comes to offering a good respondent experience on mobile devices.
Poor respondent experience can have disastrous consequences, impacting your survey response and completion rates, and even impacting the data quality of your study.
So how can you follow best practices for mobile surveys?
Build on a Mobile-First platform
By using Pollfish, you are already off to a great start. That is because every Pollfish survey question type is optimized for mobile out of the box.
Our surveys are not only optimized to fit your mobile screen, they are delivered exclusively inside mobile apps, meaning each question type is designed by app developers to mirror the experience of an app.
Pollfish also provides a mobile previewer for each question in your survey, so you can test how your question will look on a phone in real time, right inside the questionnaire builder.
<Maybe add a screenshot of the questionnaire builder here?>
Shorten your questionnaire
Because so many users prefer to take surveys on mobile devices, it is important to think like a mobile user.
Think about your app usage–do you stay in one place on your phone for a long time, or do you jump quickly from place to place? Do you often have your phone open for hours or do you use your phone in quick bursts?
Mobile phone users want to move fast. They could be on the go. They could only have a few minutes to kill while waiting in line at the bank.
That’s why it is important, when you can, to keep surveys short.
Shorter surveys get faster results and ensure full engagement with your questions from start to finish.
Our survey experts review every survey before it is published as well, so they may recommend you shorten parts of your study if they feel respondent experience may be impacted.
Keep a mobile mindset
While all our survey questions will look great on mobile, there are a few things you can do to make your questionnaire more engaging to the mobile user.
Start by favoring question types that are easier to answer on mobile phones. Multiple-choice questions that users can tap, rather than open-ended questions where typing is required makes answering easier for users. Question types like rating stars and using video can keep users engaged and motivated throughout your survey.
Keep in mind that while many survey tools that offer a “mobile survey” experience also offer matrix questions, you’ll want to verify that these have been designed for mobile distribution.
Matrix questions in a typical survey present as a table where respondents are asked to rank a series of answers. In mobile, these don’t translate into a good experience for respondents.
Ensure that your mobile matrix question utilizes vertical scrolling and doesn’t require respondents to zoom in or drag the table horizontally to see all the answer possibilities.
While more qualitative questions may be helpful to provide more context and emotion to survey respondents, mobile respondents would rather tap or swipe than type.
If you were a mobile user, which of these would you prefer to answer?
Prevent Survey Bias
One of the most important parts of building the perfect survey is reducing survey bias.
From Your Ultimate Guide To Survey Bias
Put simply, survey bias impacts data quality. By eradicating survey bias (as best you can), you build trust in the data you’ve collected.
That’s no small thing, as companies across the world are using consumer insights data to inform everything from content creation to product timelines to strategic planning.
So how do you remove bias from your questionnaire?
Well, for starters, it is important to remember that preventing all survey bias is impossible.
That’s right. We are all biased. We have unconscious thoughts that cause us to subtly indicate our true feelings to those around us. And that applys to writing survey questions as well.
The key is to do everything you can to remove the most pernicious forms of bias from our surveys so we can ensure top-quality data.
With this section, I’ll take you through a few of the more common ways to avoid bias in your questionnaire.
Remove Double-barreled questions
Double-barrelled questions happen when researchers blend two questions into one, and then allow for only one answer. This creates a fallacy for the respondent and often results in incomplete or biased answers.
Can you tell which question is biased here?
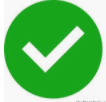
While this question may not appear to cover as much ground as it’s counterpart, the other question here doesn’t allow the respondent to choose different answers for the Pool and Bar. It is always better to split double-barreled questions into two separate questions to ensure specificity.
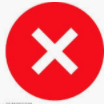
This question doesn’t allow respondents the ability to select different ratings for the pool and bar. Even if you consider the pool and bar to be the same, a respondent may not. Double-barreled questions provide incomplete answers. You’ll risk losing critical consumer insights. Make sure you split them in two.
Avoid Assumptions
Depending on how you write a question, you could be assuming things of the respondent, or even forcing them to give an answer they don’t believe.
One way researchers fall into this trap is by asking loaded questions. Loaded questions make multiple assumptions about a person and then force them to answer a question based on those assumptions.
Can you spot the Loaded question?
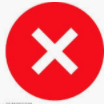
This question presupposes the respondent likes attending baseball games, because they have to fit the premise of the question in order to answer. Before you can ask this question, you must ensure your respondents like attending baseball games. You can do this by asking such a question and then adding skip logic so that only those who have previously attended baseball games will be shown this question.
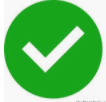
Before a respondent can answer a question about snack preferences at a ballpark, you have to first establish that your respondents attend or have attended baseball games. You can ask the above question and apply skip logic so only those who fit the premise of your intended question are shown it.
Don’t Use Biased Language
Similar to Loaded questions, biased language (sometimes called Leading questions) pushes respondents towards one response or the other.
This removes the impartial nature of a survey and influences respondents, leading to poor data quality.
Can you spot the Leading question?
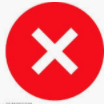
The words “new” and “old” bias the respondent towards saying the newer design is easier to use. You need to ask questions from a neutral position and do your best to avoid biased language that lead respondents towards one answer or another.
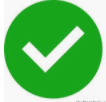
By asking, simply and directly, which design is easier to use, without letting the respondent know which is newer or older, you ensure that you remove bias. By adding responses like Not Sure or No Difference, you open up the question to additional answer possibilities for a broader range of respondents.
Do Your Best To Include All Available Choices
As a general rule, you want to avoid forcing respondents to give an answer they don’t really mean.
So when you are writing a closed-ended survey question with a finite answer pool, make sure you think hard about additional answer possibilities that may not come immediately to mind.
Once you have done that, there are two additional ways you can reduce bias on these kinds of questions.
The first is to add an open-ended “Other” field, where respondents can include any additional choice you may have forgotten. If there is enough consistency in responses, you may even be able to manually quantify the data. If not, you have still provided some much-needed context to the current answer choices.
If you want to stick with your closed-ended question type, you can make it clear in your instructions that respondents are to select the answer closest to what they think. This is imperfect and provides a tough-to-quantify variable to your data. But it also will keep respondent experience and completion rates up.
Market Research Terms Glossary
Contents
Market Research Terms Glossary
You can’t become a market research expert without learning the lingo. That’s why we created the Pollfish Market Research Terms Glossary.
This alphabetical listing of key market research terms, concepts and ideas has been segmented into four sections: Market Research Terminology, Survey Design & Question Types, Survey Bias and Pollfish Features.
For basic research concepts, check out the Market Research Terminology section. Survey Design & Question Types gives simple definitions of the different types of surveys and questions you can build with Pollfish. The Survey Bias section takes you through the ways you can bias your results. And the Pollfish Features section gives you definitions of Pollfish-specific tools and features so you’ll always know what’s going on.
Check it out!
Section 1: Market Research Terminology
The more you read about market research, the more surveys you build and the deeper you sink into the world of consumer insights, the more you translate the language surrounding it.
Here, we define some basic terms that you will need to have an understanding of to become a market research expert.
A
Agile market research
An approach that values numerous small experiments over a few large bets, rapid iterations over big-bang campaigns and responding to change over following a plan.
C
Complete
A fully completed survey that has been screened for bias, insufficient responses and bots.
Completion Rate
The rate at which surveys are completed as compared to the number of surveys started by respondents. To calculate completion rate, divide the number of completes by the number of starts.
Consumer Insights
Valuable information on the preferences, opinions, habits and emotions of your most valuable customers. Consumer insights usually encompass insights related to a product or service.
Control Group
Survey participants can be split into two groups–an experimental group, exposed to a product or service, and a control group that is neutral. A common example is ad effectiveness testing, where researchers can track a respondent’s exposure to an ad through cookies. They then split those who have viewed the ad and those who have not into separate groups, asking the same questions to see how responses differ.
Cost Per Complete
The price you pay per completed survey. This calculation is based on certain factors of your survey, including the number of screening questions, quotas, demographic/geographic filtering and more. Learn More >
Cross Tabulation (Crosstab)
A feature of your survey platform that presents data in a table with rows and columns, designed to help researchers observe two or more variables at the same time. Crosstabs are useful when you want to divide your respondents into subgroups to see how a dependent variable changes the results.
D
Data
Any information collected by your survey, along with any outside information collected, observed, generated or created in service of your research goals.
Data Cleaning
Removing unqualified, biased or incomplete responses from a survey. This process improves data quality and protects against survey bias. Learn More >
Device ID
An individually assigned ID given to a Respondent’s device to differentiate them from other respondents. Many survey platforms who deliver surveys via mobile devices will collect or create Device IDs. At Pollfish, these IDs are passed to us from the mobile app publishers where we deliver your survey. This ID, along with demographic information respondents give on their first survey, are collected and used for targeting and filtering purposes in the future. Learn More >
DIY market research
Market research conducted using a self-service platform, as opposed to partnering with a market research agency or research consultant. Most DIY market research is conducted in-house to avoid the speed and cost limitations of working with outside entities. Learn More >
Drop-Offs
When a respondent begins a survey and doesn’t complete it. These are also called Starts. Drop-offs are not counted as completes and, therefore, you will not be charged for them.
E
Engagement
Engagement has many meanings. It can refer to a high degree of focus and interest in stimuli. In digital marketing and technology, engagement often refers to metrics surrounding use of platforms, content features or apps (think clicks, time on page, etc). In market research, engagement refers to how users interact with your survey. Does their time spent on each question indicate they are confused or don’t understand how to choose an answer? If so, your completion rate could be impacted.
F
Feasibility Study
A feasibility study is designed to determine the likely success of a project, product or service. There are many factors that go into a feasibility study, including existing competitors, production limitations, timing, estimated pricing and more. Brands or researchers may conduct feasibility studies to determine the market interest in a new product or service, or even to help determine the feasibility of a future research project.
Fielding
Fielding refers to the distribution of the survey questionnaire. When using Pollfish, you can watch your results roll in in real time using our Results Dashboard.
I
Implicit data
Implicit data refers to information that is not provided from respondents directly, but is gathered from available data. For example, Pollfish collects location data and information on a respondent’s mobile carrier from the App publisher, not from the respondent directly.
Incidence Rate
Incidence rate is the measure for the rate of occurrence or the percentage of persons eligible to participate in a survey, based on the targeting criteria selected.
L
Longitudinal Research
Researchers performing a longitudinal study will run the same survey many times over short or long periods, in an effort to observe how the opinions, behaviors or habits of the same population change over time. The population can also be randomized to see how time impacts the questions being asked, regardless of population.
M
Margin of error
Margin of error, also called the confidence interval, is a statistical measurement of difference between survey results and the population value, expressed as a percentage. Within the survey ecosystem, the margin of error measures the difference between your survey results and how accurately they reflect the views of the overall population. Learn More >
Market research
Market research refers to the gathering of consumers’ needs, preferences, habits, behaviors and more in an attempt to better understand a company’s potential customers, brand positioning and potential interest in a product or service.
Mobile ID
Also known as the Advertising ID, this unique ID number is how mobile advertisers are able to cookie users and keep track of engagement with mobile ads. At Pollfish, we use this id in a similar way, delivering surveys to users in much the same way an app delivers ads. We use the advertising ID to ensure we don’t send the same survey to the same user more than once. Learn More >
N
Non-Probability Sampling
Non-probability sampling excludes some of the population in your sample, and that exact number can not be calculated – meaning there are limits on how much you can determine about the population from the sample. These methods include convenience sampling, quota sampling, judgement sampling and snowball sampling. Learn More >
O
Online panel
Online panels collect responses either via a fully opt-in structure, including a signup page, or start with some form of digital outreach to potential respondents who have agreed to take surveys in advance. Panelists are then recruited to participate in specific surveys, for example via email invitation to the page of the panel provider. Pollfish avoids the pitfalls of traditional online panels by asking users to take surveys while they are using apps or games in real-time, increasing data quality and reducing biased responses. Learn More >
P
Panel
A panel is a collection of potential respondents who have agreed to take a survey in advance of the survey’s fielding process. These respondents are typically promised some type of incentive in exchange for joining the panel, which would effectively pay them for their time. Learn More >
Piping
Piping allows researchers to personalize surveys by ‘piping’ an answer from a previous question into a later question. For example, you can ask a respondent their name or occupation on the first question, and then add that name or occupation to future questions to make the questions more personalized.
Population
The population is the total group of respondents who you attempted to survey. If they complete your survey, they become part of your sample.
Primary Data
Primary data refers to the data collected by researchers directly from respondents using surveys, interviews or direct observation.
Primary research
Primary research refers to the methodology of using only data collected directly from respondents, rather than relying on data collected during previous research or from some external source (government agencies, employment records, etc).
Probability Sampling
Probability sampling refers to a randomized method of respondent selection. In order to utilize probability sampling, researchers have to have a method that ensures every member of the population has an equal chance of being chosen to participate (like picking names out of a hat).
Psychographic
Unlike demographics, which explain who your respondents are, psychographics seek to explain why they do what they do. While any quantitative study, group of screening questions or even secondary location data can net you demographic data, psychographics are more often culled from qualitative studies. For example, is your respondent concerned with health and appearance? Do they enjoy socializing or are they more introverted? You can get answers to these questions from scale-based quantitative questions, but open-ended questioning or interviewing often provides more depth to these groupings. Depending on how you plan to use them, you should consider this before creating your survey. Learn More >
Public Opinion Research
Public opinion refers to the opinions of a majority of people in a certain population. Polling the public opinion requires taking as broad of a study as possible and asking direct, quantifiable questions about specific issues.
Q
Qualification rate
The qualification rate is the estimated percentage of people you expect to qualify for your survey based on your targeting criteria, screening questions and other filters.
Qualitative research
Qualitative survey questions aim to gather data that is not easily quantified such as attitudes, habits, and challenges. They are often used in an interview-style setting to observe behavioral cues that may help direct the questions. Learn More >
Quantitative research
Quantitative research is about collecting information that can be expressed numerically. Quantitative research is usually conducted through surveys or web analytics, often including large volumes of people to ensure trends are statistically representative. Learn More >
Questionnaire
Your questionnaire is the list of questions you plan to ask your respondents. There are many different types of survey questions you can ask, depending on your survey goals. Learn More >
Quota
Quotas are limits you can set for the number of responses your survey collects from a particular group. They can be set across the entire survey or on a given question or segment. Unlike weighting, which ties your quotas to existing data sets like a national or local census, quotas can be chosen by the researcher to match the goals of your survey. If, for example, you’d like to survey a population that is 75% female, you can do that.
R
Respondent
A respondent is a person who meets your targeting criteria and completes your survey in full.
Response Rate
The response rate is the percentage of the total targeted population who responded to your survey. Learn More >
S
Sample
Your sample refers to the respondents who matched your targeting criteria and completed your survey.
Sample size
Your sample size is the number of completes your survey receives. Learn More >
Secondary data
Secondary data refers to data that has been collected outside of the bounds of a researcher’s survey, but which the researcher can use to add context. For example, DMV records, national census records and other government information can be used for things like weighting and quotas, or just to show the disparity between perception and reality within a studied population.
Secondary research
Secondary research refers to the summary or synthesis of existing research towards a new research goal. In this practice, previous primary research projects are used as sources.
Segmentation
Segmentation studies seek to separate larger audiences into smaller segments based on similar tastes, interests, perceptions and other secondary factors like education, employment or lifestyle.
Statistical significance
A measurement to quantify whether a result is just due to chance or due to some significant factor. Getting a survey that is not statistically significant comes from sampling error. That’s when your sample doesn’t accurately reflect the population of your survey and, therefore, may cause skewed results. There are two things to contend with when trying to remove sampling error: sample size and variation. The larger the sample size, the less chance there is in the result so that reduces sampling error and increases significance. Controlling for variability of your sample also can impact sampling error–the more variability in your sample, the more prone to error the study will be.
Survey
In its most basic form, a survey refers to the questionnaire–delivered either in person or online–that a researcher administers in service of a research study. When using Pollfish and other online survey platforms, a survey refers to the targeting you select, the questionnaire you create and the results files created once you run your survey. Each survey’s full info is grouped together in your account dashboard.
T
Targeting
Targeting refers to the criteria you select to screen potential survey respondents. Once targeting is selected, a population is created and your survey is delivered.
Tracking Study (Tracker)
Tracking studies use the same questionnaire, delivered over time, to track brand awareness, monitor customer satisfaction, study consumer interest in new products or services, analyze the effectiveness of advertising creative and more. Tracking studies may be delivered to the same populations (to gauge how perceptions of the same group changes as time goes on) or different populations to view time as just one factor impacting shifting perceptions.
Section 2: Survey Design & Question Types
When creating your survey design, it is important to start with the type of survey you want to run. Understanding the small but significant differences between survey types is a great first step.
Here, we want to review the different types of studies you can run with Pollfish, as well as the ideal question types for each one.
A
Ad Absorption
A study designed to show the effectiveness of certain advertising creative. These studies tend to use narrow audience targeting screening questions and even control groups to determine if new ad creative is having statistically significant impact on user experience or understanding of a product, brand or service. Learn More >
Attitude & Usage Testing
A study that aims to understand the available market for a product or service. This typically involves things like market sizing, general understanding of the product category, questions understanding brand decisions, gathering targeting information and more.
Audience Profiling
Profiling is a process of collecting demographic information to define an audience or population. Researchers may need to conduct surveys of a population to determine if there are enough available survey takers for the demographic or other targeting they want to run. At Pollfish, we use a rolling profiling model to keep our audience up to date. We also collect most of the demographic data you will need up front, including demographic, gender and mobile usage data.Learn More >
Audio question
A question that requires the respondent to listen to an audio clip in full before answering.
B
Brand Awareness
Brand awareness is the extent to which consumers are familiar with a brand, the extent to which the brand’s intended perception matches reality and the extent to which a company’s brand is helping or hurting sales.
C
Closed-ended question
Closed ended questions are those that offer a limited selection of answers to choose from, such a single or multiple-selection question, matrix, or scaling question type. Learn More >
Competitive Analysis
Competitive analysis testing helps you better understand how your potential customers are discovering your competitor’s product, feature preferences, user behavior and more. Learn More >
Concept Testing
The process of testing a big idea, concept testing allows you to run ideas past a sample of your target market before producing them. These ideas can be new logos, new product ideas, new ad campaign ideas and more.
Content Creation Surveys
Conducting surveys can reveal gaps in news coverage that can help digital media brands develop a unique editorial voice. By surveying outside of existing readers to prospective readers and even competitive readers, digital media companies can gauge interest in, and existing knowledge of–certain topic areas. Learn More >
Creative Testing
A test allowing a creative team to gauge the effectiveness of their work at conveying the feelings and emotions they were aiming for. This includes logo testing, ad creative testing and more. Creative testing may ask questions about brand effectiveness, gauge tone and consumer enjoyment and gauge the likelihood of someone to purchase before and after viewing creative assets.
D
Description question
A question in your questionnaire that contains a basic description. This question type is usually used to provide directions to the respondent. Learn More >
I
Image question
A survey question requiring respondents to look at and respond to an image or a group of images.
L
Likert Scale
A Likert Scale is typically a 5 or 7 point scale that asks a respondent to express how much they agree or disagree with a statement. Learn More >
Logo Testing
A type of creative testing focused on changes, updates or just current opinions of a brand or product logo. This type of survey may contain questions gauging how appealing, authoritative or on-brand a logo is, or even reveal possible changes to a brand logo and ask potential customers to weigh in. Learn More >
M
Market Analysis
Studies that focus on the size, scope and potential of a market. Before launching a new product, companies may want to figure out the size of the potential market by broadly surveying interest for that product across key areas and demographic groups. This helps companies determine how large a product rollout may be needed.
Matrix multiple selection question
A matrix question asks respondents to make selections for multiple options on a scale. A matrix multiple selection question lets you select multiple responses for a single option. For example, a matrix may contain elements of a hotel, asking respondents to rate elements like the pool or the lobby bar using a variety of potential responses like “Clean” or “Fun.” A multiple selection matrix will allow you to check all descriptions that apply. Learn More >
Matrix single selection question
A matrix single selection question will ask you to select one answer per option on a scale. For example, a matrix may contain elements of a hotel, asking respondents to rate elements like the pool or the lobby bar using a variety of potential responses. Because responses are single-selection, researchers will often ask something more definitive, like “How likely were you to use these different elements of our hotel?” And ask for a scale of 1-5. Learn More >
Multiple selection question
Multiple selection questions contain a list of options and ask respondents to select all that apply. For example, the question “what kind of music do you like?” will contain a list of music types so respondents can select all types they prefer. Learn More >
N
Naming Tests
Studies concerning the names of things–new product names, new website URLs, new brand names or even the name of a new film or TV show–naming tests help reveal potential perception issues that can occur. For example, does one title effectively convey what the product or service is? Does the name defy expectations and surprise the user?
Net Promoter Score (NPS)
Net Promoter Score is a widely used customer/consumer experience test that asks a simple question: on a scale of 1-10, how likely are you to recommend this business, brand, product or service to a friend or colleague? Respondents who rate 0-6 are detractors, 7-8 are considered passive and 9-10 are considered promoters. Learn More >
NPS Survey
An NPS Survey asks the Net Promoter Score question to a specified target population. This allows companies to gauge how well they are doing on the NPS scale with their specific target customer or potential customer. Learn More >
Numeric open-ended question
An open-ended question that requires a numeric answer. For example, researchers may ask how much money you’d potentially pay for a product or service. Learn More >
O
Open-ended question
Open-ended questions do not require the respondent to select from a specific list of responses, but instead asks them to type their response into a text box. Open-ended questions, therefore, are less definitive, seeking to gather more qualitative responses, getting at the feelings and broader opinions from the respondent in their own words. Learn More >
P
Package Testing
Studies that provide consumer feedback on product packaging. Would you be more or less likely to buy a product with new packaging? Does the packaging effectively convey what is inside? How do your potential customers feel when they look at your new packaging?
Pre/Post Studies
A pre-post study examines whether participants in an intervention improve or become worse off during the course of the intervention, and then attributes any such improvement or deterioration to the intervention.
Product / Market Fit
Product/Market fit is a the degree to which a particular product meets the demands of the market it is in. Have you created the minimum viable product for your market, that solves a problem or need that exists? Product/Market fit studies aim to survey early adopters or potential customers to see if the product meets previously identified criteria for satisfaction within a market.
Product Testing
A final test of a product, product testing gives users access to a prototype and seeks to identify opinions–positive or negative–of a product before it goes to market.
Q
Qualitative survey question
Qualitative research focuses on personalized behavior, such as habits or motivations behind their decisions. This can be gathered through contextual inquiries or interviews to learn more about feelings, attitudes, and habits that are harder to quantify but offer important additional context to support statistical data. Learn More >
Quantitative survey question
Quantitative survey questions are those that can be expressed numerically, meaning they require respondents to select from a pre-selected list of potential responses. Learn More >
R
Ranking question
Similar to a single-selection matrix, ranking questions ask users to rank different elements of a product or service on a numerical scale. Learn More >
Rating stars
A question where respondents answer using a scale of a number of stars. Learn More >
S
Screening question
Screening questions help researchers ensure they are getting the exact sample they want using filtering that falls outside of available targeting criteria. For example, if you only want to include people in your survey who have seen the movie Pulp Fiction, your screening question would ask potential respondents if they have seen the film, and screen them based on their answers. Learn More >
Single selection question
A question providing a list of potential responses, from which respondents may choose one answer. Learn More >
Slider question
A question where respondents answer by dragging a button across a slider to provide a rating. Learn More >
U
Usability Testing
Usability testing aims to test user experience changes on real users to determine if the experience has been positively or negatively impacted by any changes. Unlike more traditional product testing, usability testing usually focuses more on design changes and more commonly relates to app or software development.
UX Testing
Any testing related to the overall user experience of a product (usually app or software). UX testing tends to be more holistic than Usability testing, running qualitative and quantitative studies of each phase of the user journey.
V
Video question
A question where respondents much watch a video in its entirety before answering.
Section 3: Survey Bias
One of the most important factors in DIY survey creation is avoiding bias. Survey bias reduces data quality, threatening the integrity of your consumer insights.
We have gone in-depth on ways to root out survey bias here. But to fully understand the different types of bias you may have to contend with, here are some helpful definitions.
A
Acquiescence Bias
Also referred to as “yea-saying,” Acquiescence bias occurs when respondents are overly agreeable, to the point of contradicting themselves. You can avoid this by limiting the number of questions asking respondents to simply agree or disagree, as these statements often result in over-agreement. Learn More >
Alternators
Alternators are survey takers that fill in responses in familiar patterns, simply alternating responses but not engaging with the questions. You can avoid this by mixing in some open-ended, audio or video questions. If responses appear to be biased, Pollfish will remove them from your sample. Learn More >
D
Double-Barreled Questions
Double-barrelled questions happen when researchers blend two questions into one, and then allow for only one answer. The respondent must agree in total with a statement they may only partially believe, creating incomplete or biased responses. For example, “Did you find the product interesting and helpful? Yes or no?” Learn More >
E
Extreme Responding
If given the option, some respondents will select only the most extreme option. Make sure to mix up your question types. If you have several scales or rating questions, make sure to mix in some others that require a bit more attention. Learn More >
L
Leading Question
Leading questions use biased language to influence respondents in a direction. Keep questions as simple and direct as possible, taking care to remove any editorializing adjectives that may betray a preference towards one response or another. Learn More >
Loaded Question
Loaded questions make assumptions. For example, asking “what kind of candy do you get when you go to the movies?” assumes the respondent goes to the movies, eats candy and gets candy at the movies. This creates a biased environment where the respondent may not be able to answer accurately. Learn More >
O
Order Bias
The order you list question responses in a quantitative survey question can bias respondents towards choices higher on the list. If your survey platform has the ability, use answer shuffling to remove the potential for this bias. Learn More >
P
Prestige Question
Inserting prestige into a question by citing an expert opinion one way or another biases the respondent towards the expert’s opinion. For example, “Doctors say smoking causes cancer. Do you agree?” or “Do you support the President’s policy on Zimbabwe?” Learn More >
S
Self-Selection Bias
This occurs when respondents are allowed, through whatever means, to opt out of taking a survey based on its content. This biases the results by oversampling people with a previous interest or overly polarized opinion on a given topic. Learn More >
Social Desirability Bias
This occurs when respondents will attempt to discern what the researchers want them to say and respond accordingly. This often occurs when participants see the brand or topic ahead of time and try to give answers the company or researcher may want to hear. Avoid exposing this information, or asking any leading or loaded questions that may betray an interest. Learn More >
Speeders
Respondents who complete surveys far too fast to have actually read the questions or truly contemplated answers. Pollfish removes these respondents through AI and machine learning algorithms. Learn More >
Stereotype Question
When researchers infuse bias into survey questions, they open the potential for respondents to agree with or defy that bias. If, for example, a survey question reminds respondents that gender stereotypes exist around math, that question may have planted a seed in the mind of respondents that changes responses. Avoid this type of language at all costs. Learn More >
Straight-Liners
When respondents answer using the first available choice for every question. Respondents answering in familiar patterns in order to complete surveys faster will be removed from the Pollfish sample. Learn More >
Section 4: Pollfish Features
Sometimes, we get so excited about the features of Pollfish, we forget to define them all for you!
So we decided to explain them all in one place. Feel free to bookmark this section so you can return to it as you read through resources.pollfish.com.
A
Advanced branching
Also known as skip logic, advanced branching allows you to show respondents different questions based on responses to earlier questions. For example, if a respondent says they have tried your product, you can show them one set of questions and if they have not, you can show them different questions. Branching provides an alternative to screening questions. Learn More >
App Monetization
App monetization refers to the method or methods app publishers use to make money on their apps. There are a variety of app monetization strategies and platforms. Pollfish provides a way for app publishers to immediately monetize their creations by installing the Pollfish SDK and exposing their user base to Pollfish surveys. Learn More >
P
Programmatic Advertising
Programmatic advertising refers to the automated buying and selling of online advertising. Pollfish functions on a programmatic advertising model, delivering surveys in much the same way advertisers deliver ads in apps. Learn More >
Q
Quotas
If a survey must meet a set percentage of participants from an age or gender group, you can set quotas.
R
Radius Targeting
When setting geographic targeting on your Pollfish survey, you can select a city, congressional district or town. You can also use our Radius Targeting tool to select a specific radius on a map to target your survey. Learn More >
S
Skip Logic
Also known as Branching, skip logic allows you to show respondents different questions based on responses to earlier questions. For example, if a respondent says they have tried your product, you can show them one set of questions and if they have not, you can show them different questions. Skip logic provides an alternative to screening questions. Learn More >
Survey Stratification
Stratified sampling involves dividing a population into smaller sub-populations called strata. There are many ways of accomplishing this, including balancing using predetermined quotas before you run your survey, or weighting your results after the fact. Learn More >
W
Weighting
A type of stratification where quotas–often pegged to pre-existing data like a local or national census–are used to weight respondent pools to more accurately reflect the population being surveyed. With Pollfish, you can weight your survey results to the local or national census (where available) with the push of a button. Learn More >
Survey Bias: Your Ultimate Guide To Reducing Bias And Increasing Data Quality
Contents
Survey Bias: Your Ultimate Guide to Reducing Bias and Increasing Data Quality
Survey bias is a broad term. It encompasses all parts of a survey that can have bias. And, in case you didn’t know, there are a lot of trouble spots in the average survey.
But not to worry. Once you know what to look for, and have the right tools to root it out, survey bias will quickly become a thing of the past for your market research team.
But first things first: why should you care?
Put simply, survey bias impacts data quality. By eradicating survey bias (as best you can), you build trust in the data you’ve collected.
That’s no small thing, as companies across the world are using consumer insights data to inform everything from content creation to product timelines to strategic planning.
But where to begin?
Disclaimer: Preventing All Bias Is Impossible
Before we go any further, we should clear something up: no survey is 100% free of bias. Things like the incentives or influences of the questionnaire writer or the words used in various forms of sampling bias can negatively affect results when a questionnaire is distributed to hundreds of users.
Not all bias is preventable, but there are a few key areas and best practices you can use to prevent bias in your survey.
Once you have done everything you can to ensure your survey is taken from a representative sample, has a variety of unbiased questions, and is delivered to real consumers, you will be ready to get great consumer insights.
Let’s start with the basics.
The Different Types of Survey Bias
Survey bias falls into three categories: Sampling Bias, Questionnaire Bias, and Survey Fraud. There are other kinds of bias out there to watch out for (some of which we will get into at the end) but most forms of bias you should watch out for fall into these three categories.
What is Sampling Bias (and How to Avoid It)?
Sampling bias, quite simply, refers to bias that occurs in the selection of your survey respondents, also known as your sample.
There are many different types of sampling bias to watch out for.
Representation Bias
If you select your sample of a town based on a respondent’s presence at the mall, you could be excluding key members of the local population. For example, you may be over-representing healthy people of a certain age who get out of the house, people with the disposable income to shop, etc.
How to avoid it
Digital sampling methods help with this. By collecting responses from users on their phones, through social media or by email, you avoid the types of sampling bias that an in-person surveyor would contend with. If you want to take it one step further, Pollfish Survey Stratification can help (more on that later).
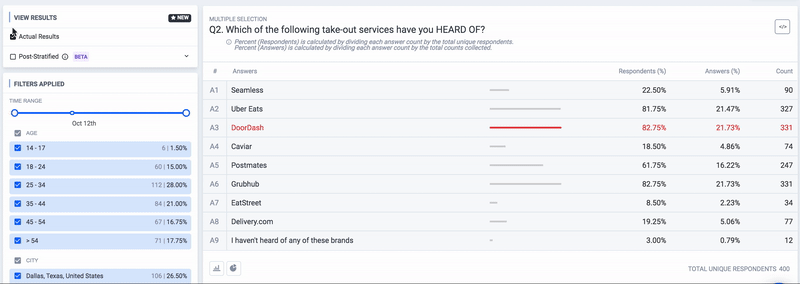
Exclusion Bias
Removing trial participants can create sampling bias. If, for example, your survey requires follow-ups but excludes original participants who have since moved out of the study area, you could skew the results.
How to avoid it
Keep initial and follow-up samples the same.
Pre-Screening Bias
This occurs when you advertise for volunteers within particular groups.
How to avoid it
One way to avoid this would be to reduce targeting on your survey collection method of choice, and instead use screening questions on your survey to screen out people who may not have the qualifications you are looking for.
Survivorship Bias
An over-emphasis on “surviving” respondents can create sampling bias. For example, doing an economic study of the business climate and only including current businesses, without including ones that failed.
How to avoid it
When doing customer satisfaction surveys, for example, it is important to get feedback not just from your current customers–who are likely satisfied–and potential customers–who don’t know how they feel about you–but from your former customers who you likely failed to satisfy. This way, you can truly know how satisfying your product or service actually is.
How to weigh your survey results in Pollfish
Other Ways to Avoid Sampling Bias
Stratified sampling
Stratified sampling is the process of dividing a population into subgroups and selecting a balanced sample audience from those groups to decrease the sampling error. These populations are usually large and diverse. Because random sampling from a given audience can be unbalanced, stratified samples apply weights to audience characteristics against a control group to ensure that the responses are representative of a given population.
Examples
- If you wanted to survey the population of Dallas, TX, you could weight your survey sample audience demographics against census data for Dallas to create a more accurate reflection of the demographic makeup of that city.
- If you were conducting a political survey and needed representation of various minority groups (such as race or religion) that may not appear in a random sample, stratified sampling could ensure that some members of those groups are included, and their responses adjusted to present a proportional segment of the population as a whole.
Quotas
Quotas are another way to weigh an audience and reduce sampling bias, but instead of applying a weighted number to audience characteristics to estimate proportionality to the population, quotas fix the proportions of an audience so that responses are collected from people who fit the exact criteria. While they typically take longer, quotas offer more precise data. They’re better used for a smaller sample population where the weighted demographics are known.
Examples
- In a survey for a magazine where readership was known to be 80% female, quotas to survey an 80% female audience would be used.
- If a product is geared towards millennials, age quotas might be used to ensure that the survey is only completed by a randomized sample of those between the ages of 25-40.
What is Questionnaire Bias (and How to Avoid It)?
When building your survey questionnaire, the way you ask a question matters just as much as, if not more than, what you are asking.
Questionnaire Bias (also known as Response Bias) can come in many forms. Where most researchers get in trouble is they bias respondents unintentionally, due to how they ask a question, what question type they use, or how they administer the questionnaire.
Self-Selection Bias
Also known as Non-Response Bias, Self-Selection Bias can occur when respondents are allowed, through whatever means, to opt-out of a survey due to its content. For example, not everyone who eats at a restaurant is forced to leave a review. Therefore, most restaurant reviews are biased toward people who had a particularly good or unpleasant experience. You also see self-selection bias when there is a knowledge gap on a topic. If the topic or subject of the survey is known and people can opt-out, the survey will get more participants with advanced knowledge of or interest in the topic or subject.
How to avoid it
You can avoid this type of bias in two ways–you can either remove the ability for participants to opt-out once they’ve seen the brand or topic of the survey, or you can keep the brand or topic hidden throughout the survey.
Acquiescence Bias
Also referred to as “yea-saying,” Acquiescence bias occurs when there are too many questions asking participants to agree or disagree. Respondents tend to overly agree, even when this causes contradictory responses.
How to avoid it
Using a variety of question types will remove this type of bias. Don’t rely too heavily on any one question style. And if you can, review responses and throw out any with deeply contradictory “yea-saying.”
Order Bias
The order in which you list selections in a single-selection or multiple-selection question biases respondents towards choices higher on the list. Similarly, responses change based on the order survey questions appear.
How to avoid it
If your survey platform can shuffle questions and/or responses, take advantage of this feature. You will instantly remove this type of bias from your survey.
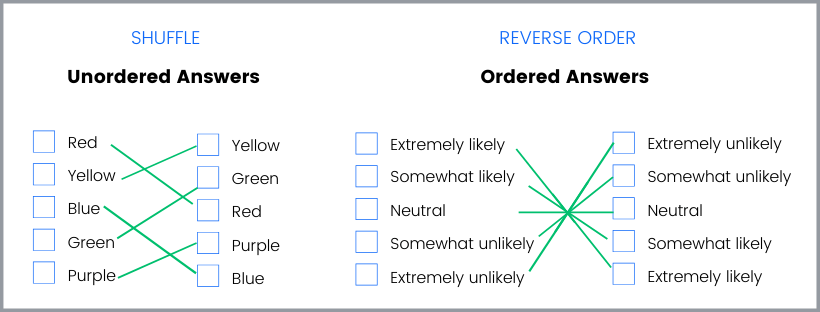
Extreme Responding
Some respondents just like extremes. So, if given the choice, they will always select the most extreme option.
How to avoid it
Like acquiescence bias, Extreme Responding is the result of too many of the same type of question. If you are going to use scales or sliders that welcome extreme responses, use other types of questions to gauge user feeling as well. This will give you a more nuanced understanding.
Social Desirability Bias
There are different forms of this bias. For example, participants sometimes believe there is a way in which they are “supposed to” act when taking part in a survey. Typically, this comes from respondents who believe in the importance of research and want to be good subjects, so they will try to discern what the researchers hope will happen in the study and give them the answers they want. Or the respondent will overly report behaviors they believe the researcher may want to hear for other reasons. The most famous example of this is in political polling in the US in 2016, where subjects hid their intention to vote for Donald Trump from pollsters.
How to avoid it
You can start by keeping the brand or topic hidden from participants, and just asking them to respond so they don’t know who the answers are for. Secondly, omit leading or loaded questions that may convey an intent or interest.
Question Types to Avoid
Loaded Questions
Loaded questions make assumptions. For example, “What is your favorite snack when you go to the ballpark?” is a loaded question, as it assumes the respondent likes sports, has been to a ballpark and has eaten snacks at a ballpark.
How to avoid them
Assume nothing. If you want to survey people who enjoy snacks at the ballpark, try a screening question or two. This will ensure you are only asking people who fit the profile.
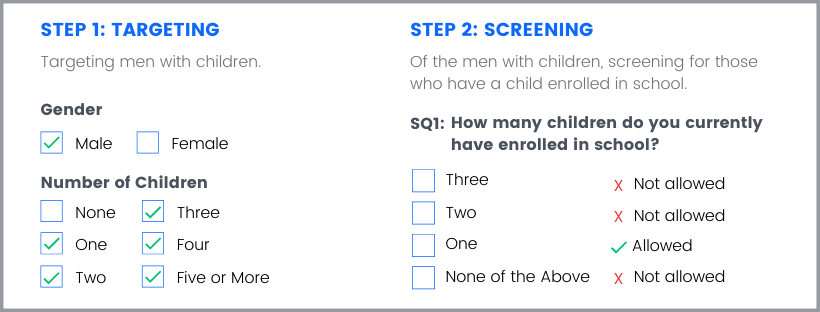
Leading Questions
Leading questions use biased language to push respondents toward a response.
How to avoid them
Keep questions simple and remove any language that may imply intent. Complimenting or insulting a product or service should be off-limits. If you can, have a friend who is unfamiliar with the subject, review your questionnaire.
Double-Barreled Questions
Double-barreled questions happen when researchers blend two questions into one and then allow for only one answer. This creates a fallacy for the respondent and often results in incomplete or biased answers. For example, “Did you find the product interesting and helpful?”
How to avoid them
Make sure each question asks for a single answer. If you have double-barreled questions, they should be split into two questions, i.e. “Did you find the product interesting?” and “Did you find the product helpful?”
How to write good survey questions
Prestige Questions
These questions insert knowledge from widely accepted experts into questions, which biases respondents to agree with the expert. For example, “Doctors say smoking causes cancer. Do you agree?” or “Do you support the President’s policy on Zimbabwe?”
How to avoid them
Avoid asking questions beyond the cognitive grasp of the average person. If your question assumes a level of knowledge, use screening questions to remove people who don’t consider themselves knowledgeable. If you provide information on a topic you are asking about, be sure to remove bias from your explanation and only ask participants to respond to the information included. When possible, use multimedia (video, audio, images), as these can be less biased than adding prestige to the information.
Negative Questions
These questions force respondents to remember a traumatic event or think about something negative. Asking about health issues, car accidents, money woes, or even divorce all qualify.
How to avoid them
If these types of questions exist in your survey, you can remove them (as they will bias respondents towards responding more negatively), or you can move them to the end.
Stereotype Questions
Researchers sometimes unintentionally stereotype their respondents. For example, reminding respondents that racial stereotypes exist around driving or that gender stereotypes exist around math ability may bias respondents towards acting more stereotypical, or trying to defy those stereotypes instead of delivering honest responses.
How to avoid them
Remove this kind of language from your survey, and don’t ask respondents to describe themselves by race, ethnicity, age, and the like, unless you have to.
Absolute / Pushy Questions
Questions with Yes or No answers can bias respondents because they don’t allow for any range in between. This may cause respondents to not answer or answer Yes when they mean Only Sometimes. For example, do you eat breakfast in the morning, yes or no? If someone doesn’t eat breakfast 100% of the time, they may answer “No” whereas another person may answer “Yes” because they do sometimes eat breakfast. The result is imprecise and biased responses. Taking this even further, some researchers will make a declarative statement and ask the respondent to agree or disagree (Spain is too hot in the summer, agree or disagree?). This presents bias because survey takers who don’t have a strong opinion will be more inclined to agree with the statement.
How to avoid them
Remove Yes or No questions from your survey. Then, read your questionnaire from a user perspective and remove all statements that may lead the user toward one response or another. Remove all declarative statements, and simply ask questions.
Ambiguous / Confusing Questions
Unclear wording, referring to earlier questions, and using indirect language are just some of the ways researchers confuse respondents. For example, “Was your breakfast not incorrect when it arrived at your table?”
How to avoid them
Read your survey questions aloud. This will allow you to hear how they may sound to a reader. You should be able to spot examples of confusing language.
What is Survey Fraud (and How to Avoid It)?
Traditionally, market researchers would assemble panels– groups of people who have agreed to take surveys. In most cases, panelists were convened in person and offered some sort of semi-lucrative incentive. Think airline miles, gift cards, and even cold, hard cash. Panelists were convened in person, preventing things like panelists from taking the same survey multiple times.
However, researchers eventually sought more randomized sampling. Enter random digit dialing, where an auto-dialer machine would call random people to collect responses for surveys. This reduced bias as respondents were selected at random and screened after the fact.
Then came the internet.
Random Device Engagement and Organic Sampling
Suddenly, research panels re-emerged. Now online, backed by the global reach and offering respondents all over the globe the ability to take surveys for pay, Panels were back with a vengeance.
This incentive structure, combined with the scale of these efforts, created an opportunity for fraud. Survey takers, looking to accumulate as many rewards as possible in as short a period as possible, would take as many surveys as possible, barely reading the questions before providing answers.
While most reputable panels seek to remove these fraudulent responses, panel participation has been on the decline, and panels often fall short of the number of respondents necessary to create a representative sample for their customers.
This requires panels to bridge this gap wherever they can find it by buying samples from wherever they can find respondents. When they lose control of the panel vetting process in-house, they have less information about the respondents, recruitment methods, or whether they are a suitable choice for the survey’s target audience.
But who are these problematic survey takers and how can you spot them?
At Pollfish, we created AI and Machine Learning algorithms to find, locate and remove these fraudulent responses (more on that later.)
If you see these types of responses popping up in your surveys, make sure you get rid of them as they don’t contribute to a representative sample.
Professionals
Sometimes companies pay people to take surveys. The amount per survey is often low, so professionals take a ton of surveys to make money as part of their overall income. These respondents will often have accounts on multiple sites, multiple aliases, etc. Their fast, haphazard movement through your survey can be disastrous to data quality.
How to avoid them
Don’t use traditional panels. Survey platforms like Pollfish deliver your survey inside mobile apps, to active consumers. Respondents receive in-app benefits, not monetary incentives.
Rule-Breakers
Rule-Breakers skip key instructions, offer incomplete responses, or contradict themselves.
How to avoid them
It can be hard to know if this is due to speeding through towards a reward or just a mistake, but either way, if there is too much rule-breaking, it is best to filter them out.
Speeders
Much like professionals, speeders can’t possibly have completed your survey accurately in the time they took. But if you aren’t tracking for this, how would you know?
How to avoid them
Set a time threshold and timestamp respondents. If you find that they moved through your survey too quickly to successfully complete it, filter them out.
Straight-Liners
Straight-liners are some of the easiest fraudulent respondents to catch. These respondents give the same or similar answers to every question. Whatever the first choice is, that’s what they select.
How to avoid them
If you spot this in your responses, or your platform can flag it, remove these respondents as there is a high likelihood their responses are fraudulent or unhelpful to your study.
Near-Straight-Liners
Sometimes survey takers know they may be filtered out and not get a reward if they answer like a Straight-Liner. So near-straight-liners will answer all but 1 or 2 questions in a straight line.
How to avoid them
Same as above. Remove.
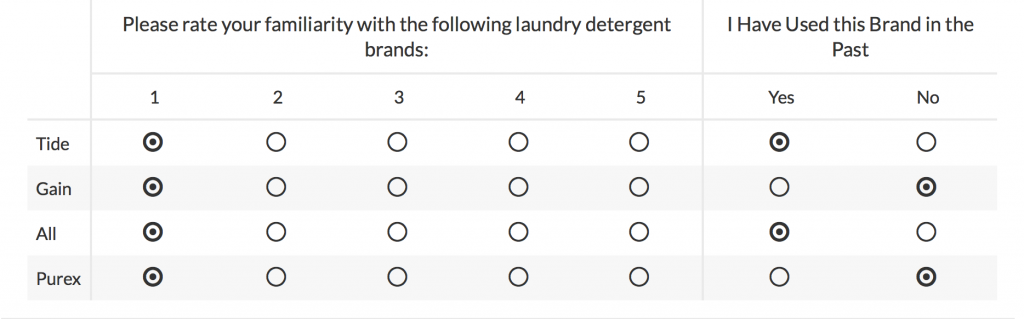
Alternators
Remember the kid in class that didn’t study for the test, so they would just fill in the bubbles on the scan sheet into diagonal lines (think A, B, C, D, C, B, A) all the way down? That kid was an alternator.
How to avoid them
These can be a little harder to spot. It would help if your survey platform could do this work for you.
Bots
For the more computer savvy fraudster, creating bots to take surveys is the easiest way to game the system. While most popular panels have installed protections against bots and have abandoned particularly vulnerable methodologies like River Sampling (using banner ads as a recruitment process without verifiable participant info), these panels may still buy respondents from companies who don’t do enough to protect against bots.
How to avoid them
Find out where your survey respondents are coming from. Make sure none of the providers in your sample are using outdated methodologies like River Sampling. If your survey platform has bot detectors and can throw out common bots, all the better.
Fake Accounts
Just like bots, survey takers will sometimes create fake accounts, allowing them to take the same survey multiple times. Most survey panels check IP addresses to protect against this, however, these protections are easily circumvented.
How to avoid them
Check if your survey platform allows accounts to take a survey more than once or repeat or restart a survey once they have started. Make sure to have a mechanism in place to filter by IP address or look for other patterns that may indicate fake accounts. Surveys that allow link sharing are more likely to suffer from repeated survey attempts, while those that use a randomization method such as RDD or RDE approach the respondents and use a unique identifier to prevent repeats.
Biased Respondents
This last one is impossible to prevent if you are working with a traditional online panel. Forums and other online meeting places allow biased respondents to find pre-recorded answers to your survey shared by other respondents. This allows them to quickly answer surveys without adding their own, natural feedback.
How to avoid them
The internet is a big place and you, as a researcher, cannot easily protect against this. You can prevent survey takers from cutting and pasting inputs, you can prevent screenshots and other measures that prevent sharing responses. But if you want to prevent this problem, it may be best to avoid survey panels all together.
Pollfish Can Help
AI / Machine Learning
Pollfish AI and Machine Learning remove bad survey takers. Read more about that here.
Individual Review
Pollfish individual review (by a person) ensures your survey follows best practices. Each survey you submit is subject to a brief (30-minute) review to ensure you have not egregiously biased your study.
Survey Stratification
Recently, Pollfish launched Survey Stratification, which allows you to tie the weighting of your survey sample to the US Census. With the push of a button, you can weight your respondents to match the Census demographics. This data is available on a national level in the US, as well as in all 50 states. It is also available across the EU and UK, as well as Iceland, Liechtenstein, Norway, and Switzerland. This feature can reduce sampling bias. Read more about this here.